The Evolution of Continuous Threat and Exposure Management (CTEM)
In a world where cyber‑adversaries continually refine their tactics, security programmes must evolve from episodic testing to an unbroken cycle of detection, analysis and remediation. Continuous Threat and Exposure Management (CTEM) represents this paradigm shift, transforming how organisations perceive and manage risk. This blog unpacks CTEM’s history—tracing its roots from early vulnerability management to today’s AI‑driven, autonomous frameworks—and explores the milestones that shaped its emergence as a cornerstone of modern cybersecurity.
1. Origins: The Vulnerability Management Era (Late 1990s–2000s)
1.1 Standardising Flaw Identification
In 1999, MITRE’s launch of the Common Vulnerabilities and Exposures (CVE) list created the first unified taxonomy for software flaws. Security teams could finally reference vulnerabilities with a consistent identifier, streamlining communication across vendors, researchers and enterprises.
1.2 Introducing Severity Scoring
By 2005, the Common Vulnerability Scoring System (CVSS) provided a numerical severity rating for CVEs. Armed with CVSS scores, organisations began running periodic vulnerability scans—often quarterly or annually—to generate “to‑do” lists of patches. Yet this static approach revealed only a snapshot of risk, and critical gaps emerged between scan cycles.
2. Expanding Attack Surfaces & the Limits of Traditional VM (2010–2020)
2.1 Cloud, IoT and the BYOD Explosion
The 2010s saw a massive increase in cloud services, Internet‑enabled devices and remote work. Asset inventories multiplied overnight, while shadow IT proliferated beyond central IT’s visibility. Traditional VM tools, reliant on fixed scan targets, struggled to keep pace.
2.2 Alert Fatigue and Static Prioritisation
As vulnerability scan results ballooned, security teams faced overwhelming alert volumes. With CVSS scores alone to guide them, organisations often remediated lower‑risk flaws first—or worse, deferred patches indefinitely—leaving critical exposures unaddressed.
3. The Emergence of Exposure Management (2020–2021)
3.1 From VM to CEM
Recognising vulnerability management’s blind spots, vendors and analysts introduced Continuous Exposure Management (CEM). Beyond software defects, CEM encompassed misconfiguration checks, attack surface discovery and basic threat intel integration. Early CEM platforms offered daily asset discovery, risk‑based prioritisation and validation workflows—yet still lacked a unified framework.
3.2 The Five‑Step Programme
Organisations practising CEM typically followed five phases—Scoping, Discovery, Prioritisation, Validation and Mobilisation—laying the conceptual groundwork for what would become CTEM.
4. Gartner Codifies CTEM (Mid‑2022)
4.1 A New Security Imperative
In July 2022, Gartner published “How to Manage Cybersecurity Threats, Not Episodes”, formally defining Continuous Threat and Exposure Management. They positioned CTEM as an integrated, programmatic approach encompassing people, processes and tools, with a focus on real‑time risk reduction rather than episodic scans or one‑off penetration tests.
4.2 Five Pillars of CTEM
Gartner’s CTEM framework mirrored CEM’s phases but emphasised continuous feedback loops:
- Scoping – Defining critical assets and business objectives
- Discovery – Automated asset and vulnerability identification
- Prioritisation – Risk‑based triage using attacker‑centric metrics
- Validation – Continuous testing and red‑team exercises
- Mobilisation – Rapid remediation and executive reporting
This formalisation galvanised vendors and adopters alike, providing a clear blueprint for implementation.
5. Industry Adoption & Refinement (Late 2022–2023)
5.1 Vendor Support
Security vendors such as CrowdStrike, NSFOCUS and Phoenix Security published CTEM playbooks, integrating attack surface management, threat intelligence, and orchestration tools into single platforms. Early adopters reported 60–80% faster risk reduction cycles, validating CTEM’s value.
5.2 Community Best Practices
Industry conferences and working groups began sharing lessons learned—stress‑testing CTEM pipelines, refining risk scoring algorithms and standardising reporting metrics to satisfy both technical teams and board‑level stakeholders.
6. The AI‑Powered Frontier (2023–Present)
6.1 Autonomous Agents and Agentic AI
By 2024, the rise of AI Agents and Agentic AI introduced autonomous sub‑systems capable of continuous reconnaissance, threat correlation and remediation orchestration. These agents:
- Scan cloud APIs, DNS records and dark‑web feeds in real time
- Correlate vulnerability data with exploit‑in‑the‑wild intelligence
- Orchestrate patch deployments and validate fixes through automated tests
6.2 Agentic RAG: Retrieval‑Augmented Generation
Combining large language models with live data retrieval, Agentic RAG systems generate up‑to‑date vulnerability briefs, incident playbooks and executive summaries on demand. Security analysts can query these agents for:
“What’s the latest exploit for CVE‑2025‑4321 and recommended containment steps?”
…and receive a tailored, actionable response within seconds.
6.3 Measurable Outcomes
Organisations leveraging AI‑driven CTEM report dramatic improvements:
Metric | Pre‑CTEM | Post‑CTEM (AI‑Enabled) |
Mean Time to Detect (MTTD) | 45 days | 3 days |
Mean Time to Remediate (MTTR) | 30 days | 2 days |
False Positives Reduction | N/A | 50% decrease |
Cost per Incident | £400,000 | £100,000 |
7. Key Lessons from CTEM’s Journey
- Continuous > Periodic
Static scans and tests cannot keep pace with dynamic infrastructures or adaptive adversaries. - Integration Is Imperative
True CTEM unifies asset discovery, vulnerability management, threat intelligence, testing and orchestration into a single lifecycle. - AI Amplifies Human Expertise
Autonomous agents and RAG systems accelerate every phase—reducing manual toil and enabling analysts to focus on strategic threat hunting. - Executive Alignment Drives Success
Board‑level reporting and clear risk metrics ensure CTEM investments translate into measurable ROI.
8. Looking Ahead
As CTEM matures, we can expect further innovations:
- Self‑Healing Networks that automatically reconfigure in response to detected threats
- Federated Threat Intelligence sharing across industry peers via secure, privacy‑preserving protocols
- Explainable AI frameworks that demystify prioritisation decisions for auditors and regulators
The history of CTEM demonstrates a relentless march toward ever‑faster, smarter and more integrated security operations. By embracing its principles—and harnessing AI‑powered automation—organisations can transform security from a sporadic burden into a continuous, strategic enabler of trust and growth.
Self‑Healing Networks: Autonomous Reconfiguration to Counter Threats
As cyber‑threats grow in sophistication and pace, static network architectures struggle to contain, isolate or remediate attacks quickly enough. Self‑healing networks represent the next evolutionary step—dynamically adapting topology, policies and controls in real time to neutralise threats with minimal human intervention. Below, we explore how self‑healing networks operate, examine practical examples, and consider key benefits and implementation considerations.
1. What Is a Self‑Healing Network?
A self‑healing network continuously monitors its own health and security posture, then automatically applies countermeasures when anomalies or intrusions are detected. Core characteristics include:
- Real‑Time Telemetry: Aggregation of network flows, device health metrics and security alerts.
- Policy‑Driven Automation: Pre‑defined rules and AI‑driven policies govern how to re‑route traffic, quarantine segments or adjust firewall settings.
- Closed‑Loop Orchestration: Detection tools (e.g. IDS/IPS, anomaly‑detection ML models) trigger responses in network controllers (SDN controllers, firewalls, microsegmentation engines).
2. Key Mechanisms & Technologies
Mechanism | Description |
Software‑Defined Networking | Centralised SDN controllers can modify routing tables, VLAN assignments and QoS policies on the fly to sidestep compromised nodes. |
Microsegmentation | Dynamic creation of granular network zones; when a threat is detected, affected workloads are quarantined automatically. |
Adaptive Firewall Rules | Next‑gen firewalls adjust rule sets in response to observed malicious traffic patterns, blocking lateral movement. |
Intent‑Based Networking (IBN) | High‑level business intents (“isolate breached segment”) are translated into low‑level device configurations without manual rule‑writing. |
3. Practical Examples
- Automated VLAN Isolation
- Scenario: An IDS flags unusual east‑west traffic from a server hosting sensitive data.
- Response: The SDN controller moves the server into an isolated VLAN, and updates ACLs to permit only essential management traffic.
- Outcome: Containment within seconds, preventing lateral movement.
- Dynamic Traffic Rerouting
- Scenario: A DDoS attack targets a public‑facing application.
- Response: The network shifts legit traffic through scrubbing centres while diverting suspect packets to sinkholes.
- Outcome: Service continuity with minimal human orchestration.
- On‑The‑Fly Microsegmentation
- Scenario: A compromised developer workstation attempts SSH access to production servers.
- Response: Microsegmentation policies automatically restrict that workstation’s network segment, blocking all non‑approved flows.
- Outcome: Threat neutralised before access escalation.
4. Benefits for the Business
- Reduced Mean Time to Contain (MTTC)
Self‑healing actions can isolate or reroute traffic within seconds, slashing containment times from hours to minutes. - Optimised Operational Costs
Automation reduces manual interventions by network and security teams, freeing experts for strategic tasks. - Enhanced Resilience
Networks automatically adapt to maintain service availability even under attack. - Improved Compliance
Automated policy enforcement ensures continuous adherence to segmentation and access control mandates (e.g. PCI‑DSS, GDPR).
5. Implementation Considerations
- Define Clear Policies & Intents
- Establish high‑level security intents (e.g. “quarantine anomalous hosts”) and map them to concrete orchestration workflows.
- Integrate Telemetry & Intelligence
- Ensure network controllers ingest rich telemetry from SIEM, IDS/IPS and AI‑based anomaly detectors.
- Start Small & Iterate
- Pilot self‑healing on less critical segments (e.g. dev/test environments), refine policies, then expand gradually.
- Maintain Human‑in‑the‑Loop
- For high‑impact actions (e.g. blocking core services), configure automated alerts requiring analyst approval based on severity thresholds.
- Test & Validate Continuously
- Use regular “chaos‑engineering” drills to verify that self‑healing actions perform as expected without unintended side‑effects.
6. Integrating with CTEM
Self‑healing networks form a natural extension of the CTEM Respond & Remediate phase:
- Detection: AI Agents and RAG‑powered systems identify anomalies or confirmed incidents.
- Decision: Agentic AI evaluates risk scores and selects appropriate self‑healing playbooks.
- Orchestration: SDN controllers, microsegmentation platforms and firewalls execute network reconfiguration.
- Validation: Automated penetration‑testing sub‑agents ensure network changes correctly contain or mitigate the threat.
By embedding self‑healing capabilities, organisations can close the loop from detection to remediation in near real time—fulfilling CTEM’s promise of continuous, autonomous defence.
Federated Threat Intelligence Sharing via Secure, Privacy‑Preserving Protocols
In an interconnected ecosystem of suppliers, partners and industry consortia, no single organisation possesses full visibility into emerging threats. Federated threat intelligence sharing enables multiple entities to collaboratively exchange actionable threat data—such as Indicators of Compromise (IOCs), Tactics, Techniques and Procedures (TTPs) and attack patterns—without exposing sensitive internal details. By leveraging privacy‑preserving protocols, federated models balance the imperative for collective defence with the need to protect proprietary information and comply with data‑protection regulations.
1. Why Federation Matters
- Broader Coverage: Aggregates diverse telemetry from different network topologies and business domains, improving early detection of novel threats.
- Reduced Blind Spots: Mutual sharing fills visibility gaps—an attack spotted by one organisation can alert peers before widespread exploitation.
- Trust‑Driven Collaboration: Formalises sharing relationships under agreed policies, ensuring participants contribute equitably and benefit fairly.
2. Core Privacy‑Preserving Techniques
Technique | Description |
Secure Multiparty Computation | Enables parties to jointly compute functions (e.g. aggregate IOC counts) over their private datasets without revealing raw data. |
Homomorphic Encryption | Allows encrypted threat intelligence to be processed—such as pattern matching or frequency analysis—without decryption. |
Differential Privacy | Introduces carefully calibrated noise into shared metrics or statistics to prevent reverse‑engineering of underlying data. |
Federated Learning | Trains machine‑learning models (e.g. anomaly detectors) across multiple datasets on‑site, sharing only model updates, not data. |
3. Protocols & Standards
- STIX/TAXII Federation
Organisations operate local TAXII servers and exchange STIX bundles of threat artefacts, enforcing fine‑grained access controls and traffic encryption (HTTPS/TLS). - MISP Federation
The Malware Information Sharing Platform (MISP) supports event‑level sharing with synchronisation policies, automated transformation rules and privacy‑preserving tags (e.g. ORG‑ONLY, TLP). - OpenDXL & Event‑Driven Frameworks
Cisco’s OpenDXL and similar message‑bus architectures circulate real‑time indicators via encrypted channels, with policy agents filtering data based on group membership. - OASIS Trust Frameworks
Emerging standards such as OASIS’s STIX‑SHARE define trust bundles and dynamic membership revocation, ensuring only authorised participants receive sensitive updates.
4. Practical Implementation Steps
- Define Governance & Trust Policies
- Establish a lightweight Data Sharing Agreement (DSA) that specifies permitted data types, retention periods and liability clauses.
- Assign a Trust Anchor or Certificate Authority to manage participant authentication.
- Deploy Federated Nodes
- Stand up local STIX/TAXII or MISP instances within each organisation’s secure enclave or private cloud.
- Configure synchronisation schedules and metadata‑only sharing for highly sensitive artefacts.
- Enable Privacy Controls
- Apply differential‑privacy algorithms to aggregated threat metrics (e.g. attack frequencies).
- Use homomorphic encryption or secure enclaves (e.g. Intel SGX) for on‑the‑fly encrypted queries over shared datasets.
- Automate & Orchestrate
- Integrate the federation layer into the CTEM Discover & Assess phase: shared IOCs feed directly into vulnerability scanners and SIEMs.
- Leverage Agentic RAG agents to retrieve contextualised intelligence from the federation network and generate executive summaries.
- Monitor & Audit
- Track participation metrics (volume of contributions vs. consumption), enforcing “give‑to‑get” fairness.
- Audit access logs and perform periodic reviews to ensure compliance with the DSA and regulatory requirements.
5. Business Impact & Strategic Benefits
- Accelerated Detection: Early warnings from peer‑reported incidents reduce dwell time by as much as 40%.
- Cost Efficiency: Shared infrastructure and distributed processing lower per‑organisation investment in threat‑hunting tools.
- Regulatory Alignment: Privacy‑first sharing aligns with GDPR, PCI‑DSS and other data‑protection mandates, mitigating legal risk.
- Strengthened Ecosystem Resilience: By pooling insights, entire supply chains become more robust against coordinated or emerging threats.
6. Insights
Federated threat intelligence transforms isolated defence postures into a collective shield, enabling entities to outpace adversaries through shared visibility. When underpinned by secure, privacy‑preserving protocols—such as secure multiparty computation, homomorphic encryption and federated learning—organisations can collaborate confidently, safeguarding both proprietary data and the wider digital ecosystem. Integrating federated sharing into your CTEM framework not only enhances detection speed and accuracy but also fosters a culture of mutual trust and shared responsibility across industry peers.
Demystifying Prioritisation: Explainable AI Frameworks for Auditors and Regulators
As Artificial Intelligence (AI) systems increasingly underpin critical risk‑management and security decisions—such as vulnerability prioritisation, incident triage or threat scoring—stakeholders demand clear, auditable justifications. Auditors, regulators and non‑technical executives require transparency into why a model deems one finding more urgent than another. Explainable AI (XAI) frameworks bridge this gap, translating opaque algorithms into human‑readable insights and ensuring compliance with evolving regulatory standards.
This blog explores:
- Why explainability matters for prioritisation decisions
- Regulatory drivers and auditor expectations
- Core XAI techniques and frameworks
- Integration into security workflows
- Best practices and illustrative examples
- Future directions for transparent AI governance
1. Why Explainable AI Is Essential for Prioritisation
Modern risk‑scoring models ingest diverse inputs—vulnerability severity, exploit‑in‑the‑wild signals, asset criticality and business‑impact metrics—to produce a ranked list of remediation tasks. However, black‑box models (e.g. deep neural networks or ensemble learners) can obscure rationale. Without clear explanations:
- Auditors cannot verify that the model aligns with policy or regulatory requirements.
- Regulators may deem AI‑driven decisions non‑compliant under rules such as the EU’s AI Act or GDPR’s transparency provisions.
- Executives cannot trust or champion AI outputs if they cannot see how key factors influence risk scores.
Explainable AI ensures that each prioritisation decision is accompanied by a coherent narrative—pinpointing which inputs drove the outcome and by how much.
2. Regulatory Imperatives & Auditor Expectations
2.1 Key Regulations
- EU AI Act (proposed): Classifies high‑risk AI (including cybersecurity systems) and mandates “adequate transparency” and human oversight for such applications.
- GDPR (UK & EU): Article 22 introduces a “right to explanation” for automated decisions affecting individuals—applicable if AI models handle personal data (e.g. user‑risk scoring).
- NY DFS Cybersecurity Regulation: Requires financial institutions to maintain “sufficient audit trails” for any automated controls impacting risk management.
2.2 Auditor Focus Areas
Auditors typically examine:
- Data Lineage: Are inputs sourced, versioned and quality‑checked?
- Model Governance: Was the model validated, version‑controlled and tested for bias?
- Decision Records: Does each generated prioritisation include an audit‑grade explanation?
- Change Management: Are model updates documented and re‑audited?
Explainable AI frameworks produce artefacts—such as feature‑importance reports and counterfactual analyses—that satisfy these criteria.
3. Core Explainability Techniques
Explainable AI methods fall into two broad categories:
Technique Type | Purpose | Examples |
Ante‑hoc Explainable Models | Intrinsically interpretable by design | Decision Trees, Generalised Additive Models (EBMs), Rule Lists |
Post‑hoc Explainability | Applies explanations to any black‑box model | LIME, SHAP, Counterfactual Explanations, Feature Attribution |
3.1 Ante‑hoc Models
- Explainable Boosting Machine (EBM): A glass‑box ensemble that sums simple functions of individual features, enabling direct plotting of feature effects.
- Rule‑Based Learners: Produce “if–then” statements (e.g. “IF vulnerability_age > 30 days AND exploit_score > 0.8 THEN priority = High”).
3.2 Post‑hoc Methods
- LIME (Local Interpretable Model‑agnostic Explanations): Perturbs inputs around a specific instance to learn a local surrogate model that approximates the black‑box’s behaviour.
- SHAP (SHapley Additive exPlanations): Assigns each feature a “Shapley value” representing its contribution to the prediction, based on cooperative game theory.
- Counterfactual Explanations: Identifies the minimal changes to input features that would flip a decision (e.g. “If the asset criticality were one level lower, this vulnerability would move from High to Medium priority”).
4. Frameworks & Tools for Explainable AI
Several open‑source and commercial frameworks accelerate XAI adoption:
Framework / Library | Capabilities |
IBM AI Fairness 360 | Bias detection, explainability metrics and pre‑built pipelines for fairness and transparency. |
Microsoft InterpretML | Implements SHAP, LIME, EBMs and interactive visualisations for model explanations. |
Google What‑If Tool | Visual interface for analysing model performance and generating counterfactuals directly in TensorBoard. |
Alibi | Offers anchor explanations, contrastive explanations and integrated support for anomaly detection. |
These frameworks produce visual dashboards, JSON reports or HTML artefacts that can be embedded into audit dossiers or regulatory filings.
5. Embedding Explainability into Prioritisation Workflows
5.1 Data Ingestion & Feature Tracking
- Maintain Data Lineage: Use data‑catalogue tools to tag each feature (e.g. CVSS score, exploit availability) with source, refresh cadence and quality metadata.
- Feature Store Integration: Centralise feature calculations, ensuring reproducibility across training and inference.
5.2 Model Training & Validation
- Select Interpretable Models When Possible: For moderately sized feature sets, prefer ante‑hoc learners (e.g. EBMs) to simplify explanations.
- Evaluate Explainability Metrics: Use quantitative metrics (e.g. explanation fidelity, stability) to compare approaches.
5.3 Generating Explanations in Production
- Real‑Time Attribution: For each new prioritisation decision, automatically compute SHAP values or anchor points and attach them as metadata.
- Narrative Summaries: Convert numerical attributions into plain‑English rationale (e.g. “Asset criticality contributed +0.35 to the risk score, while the absence of an active exploit reduced it by 0.12”).
- Decision Logs: Store explanations alongside model inputs and outputs in a secure, immutable audit trail (e.g. a tamper‑evident database).
6. Illustrative Case Study: Banking Vulnerability Prioritisation
Scenario: A large retail bank uses an AI model to rank patching priorities across 10,000 servers.
- Model Input Features
- CVSS Score (0–10)
- Exploit Maturity (0–1 scale)
- Business Criticality (low, medium, high)
- Regulatory Impact (e.g. PCI scope vs non‑PCI)
- Time Since Disclosure (days)
- Model Choice
- The team selects an Explainable Boosting Machine (EBM) to balance accuracy with transparency.
- Explanation Generation
- For Server A, EBM plots show:
- CVSS +0.40
- Exploit Maturity +0.25
- Business Criticality +0.30
- Time Since Disclosure –0.05
- Narrative: “Server A’s high CVSS and the availability of a mature exploit drove its priority to ‘Critical,’ offset slightly by the fact the vulnerability was only disclosed 3 days ago.”
- For Server A, EBM plots show:
- Audit Deliverables
- Model Card: Documents dataset sources, validation results, fairness assessments.
- Explanation Report: A per‑decision PDF summarising feature contributions.
- Version Control: All model and code changes tracked via Git, with audit‑grade commit messages.
When external auditors reviewed the process, they confirmed that each prioritisation could be traced end‑to‑end—from raw data ingestion through to a human‑readable explanation—satisfying both technical scrutiny and regulatory requirements.
7. Best Practices for Explainable Prioritisation
- Design for Interpretability
- Involve auditors and compliance teams early to define explanation requirements.
- Where possible, favour simpler models or hybrid architectures (e.g. rule‑based filters followed by a lightweight scorer).
- Human‑Centric Explanations
- Tailor explanation granularity: summary sentences for executives, detailed attributions for data scientists.
- Use visualisations (bar charts, waterfall plots) to depict feature impacts intuitively.
- Continuous Monitoring of Explanations
- Track explanation drift: ensure feature contributions remain consistent over time and flag anomalies (e.g. a feature suddenly dominates all predictions).
- Automate alerts if explanation patterns shift beyond defined thresholds.
- Governance & Documentation
- Maintain a central Model Registry with metadata on model versions, training data snapshots and explanation capabilities.
- Provide readily accessible Model Fact Sheets to auditors and regulators, detailing model purpose, scope, performance and interpretability.
- Secure Decision Logging
- Leverage tamper‑evident logs (e.g. blockchain or append‑only databases) to preserve the integrity of recorded explanations.
- Encrypt logs at rest and in transit to protect sensitive information.
8. Looking Ahead: The Future of Explainable Prioritisation
- Concept‑Based Explanations: Moving beyond raw features to higher‑level concepts (e.g. “financial‑data‑exposure” rather than specific CVSS sub‑scores).
- Interactive Audit Interfaces: Web‑based portals where auditors can drill down into individual decisions, toggle features on/off and view counterfactual scenarios.
- Regulatory‑Driven Standards: Emergence of ISO or NIST guidelines for AI explainability in risk management, further codifying best practices.
9. Final Insights
Explainable AI frameworks are no longer optional add‑ons—they are fundamental to embedding trust, accountability and regulatory compliance into AI‑driven prioritisation systems. By combining interpretable models, post‑hoc explanation techniques and rigorous governance processes, organisations can deliver risk‑based decisions that withstand auditor scrutiny and satisfy regulatory mandates. Ultimately, transparent AI not only mitigates legal and compliance risks but also fortifies stakeholder confidence in automated security operations.
“In a world of complex algorithms, transparency is the ultimate audit trail.”
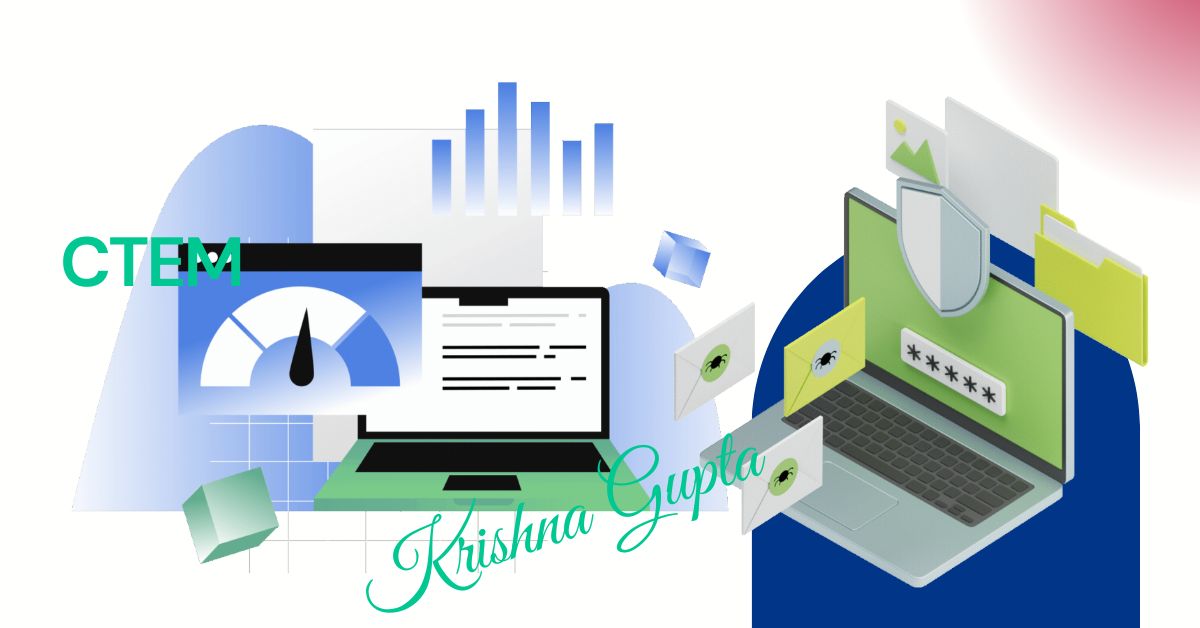
Adopting explainable AI today ensures that tomorrow’s prioritisation decisions remain both powerful and underpinned by crystal‑clear rationale.