Securing Next-Generation Biometric Systems: Defending Against AI-Powered Threats
Introduction
As India accelerates its digital transformation, the reliance on biometric identification systems is increasing across sectors—ranging from financial services to healthcare. These systems, bolstered by artificial intelligence (AI) and machine learning (ML), offer a robust and user-friendly means of authenticating identities. However, as the sophistication of these systems grows, so too does the threat landscape. Malicious actors are leveraging AI to identify and exploit vulnerabilities, posing unprecedented risks to sensitive biometric data.
This article delves into the core challenges facing next-generation biometric systems, the evolving threat vectors, and strategic approaches for mitigating these risks. By addressing these issues proactively, businesses can secure their biometric infrastructure while maintaining operational efficiency and ensuring compliance with regulatory frameworks.
Understanding the Business Context
Biometric systems are no longer limited to fingerprint or facial recognition. Modern advancements incorporate multi-modal biometrics, edge computing, and real-time analytics, enabling rapid and accurate identity verification. However, these technological leaps also open new attack surfaces that require vigilant protection.
The fusion of AI with biometric technologies introduces vulnerabilities across the entire system lifecycle, from data capture and storage to authentication and decision-making. Effective biometric security is not just a technical imperative but a business necessity. Failure to secure these systems could lead to financial loss, reputational damage, and regulatory non-compliance.
Key Technological Trends Driving Biometric Security
- Multi-Modal Biometrics: Combining fingerprints, iris scans, voice recognition, and behavioural metrics enhances accuracy but increases complexity.
- Edge Computing: Local processing on devices reduces latency but creates new opportunities for localised attacks.
- Real-Time Analytics: Continuous monitoring improves detection but can be exploited by adaptive threats.
Real-World Cyber Breaches and Incidents on Biometrics in India
Biometric authentication has become a cornerstone of India’s digital transformation, with systems like Aadhaar, facial recognition, and fingerprint-based security playing a significant role in financial transactions, identity verification, and national security. However, the increasing reliance on biometrics has also made these systems attractive targets for cybercriminals. This article delves into real-world cyber breaches involving biometrics in India, analysing their impact, the loopholes exploited, and the lessons to be learned.
1. Aadhaar Data Leaks and Breaches
Case Study: The 2018 UIDAI Aadhaar Data Leak
The Unique Identification Authority of India (UIDAI) manages Aadhaar, the world’s largest biometric database, containing personal details of over 1.4 billion Indians. Despite its security claims, Aadhaar has suffered multiple breaches over the years.
In 2018, a major data breach exposed Aadhaar details of over 1 billion Indian citizens. Reports suggested that sensitive biometric data, including fingerprints and iris scans, were being sold on the dark web for as little as ₹500. The breach was attributed to vulnerabilities in government websites and third-party service providers, allowing unauthorized access to Aadhaar-linked information.
🔹 Impact: Financial fraud, identity theft, and unauthorized access to banking services.
🔹 Lesson: Strict security protocols, including multi-factor authentication and encryption, must be enforced across Aadhaar-linked databases.
2. Biometric Bypassing in Banking Systems
Case Study: SBI and PNB Biometric Authentication Failures
State Bank of India (SBI) and Punjab National Bank (PNB) were among multiple banks that introduced biometric authentication for ATM transactions and banking services. However, vulnerabilities in biometric verification processes led to instances where cybercriminals successfully bypassed security controls.
A 2019 incident revealed that hackers used high-resolution fingerprint replicas created from stolen Aadhaar-linked biometric data to withdraw money from bank accounts without authorization. These attacks were executed using cloned fingerprint scanners and moulds derived from compromised biometric databases.
🔹 Impact: Fraudulent financial transactions, unauthorized fund transfers, and loss of customer trust.
🔹 Lesson: Banks must integrate liveness detection, AI-driven anomaly detection, and secondary authentication layers to strengthen biometric security.
3. Facial Recognition Failures and Privacy Risks
Case Study: Delhi Police Facial Recognition Errors (2020)
The Indian government has increasingly deployed facial recognition systems (FRS) for law enforcement and public surveillance. In 2020, the Delhi Police’s facial recognition system was used during protests to identify individuals. However, the system showed an alarming 30% error rate, misidentifying people and leading to wrongful detentions.
Additionally, reports surfaced about data scraping attacks on government facial recognition databases, potentially allowing cybercriminals to access and manipulate facial biometric data for identity fraud.
🔹 Impact: Misuse of personal data, wrongful arrests, and ethical concerns over surveillance.
🔹 Lesson: FRS must be audited for accuracy, and a robust legal framework must govern its usage to prevent abuse.
4. SIM Card Fraud through Biometric Exploits
Case Study: Aadhaar-Based SIM Swaps (2021)
In 2021, multiple telecom fraud cases emerged where cybercriminals exploited Aadhaar-linked biometric authentication to issue duplicate SIM cards. Attackers used stolen biometric data to bypass SIM activation security, allowing them to hijack victims’ mobile numbers. These incidents resulted in unauthorized access to banking OTPs, leading to financial fraud.
🔹 Impact: SIM card cloning, financial fraud, and personal data breaches.
🔹 Lesson: Telecom providers must enforce stricter SIM issuance policies, such as requiring multi-layer biometric verification rather than relying on a single fingerprint match.
5. Biometric Identity Theft on Dark Web
Case Study: Dark Web Sale of Indian Biometric Data (2022)
In 2022, cybersecurity researchers uncovered Aadhaar and biometric data of millions of Indians for sale on the dark web. Threat actors were offering fingerprint and iris scan records, potentially enabling criminals to bypass biometric authentication systems used in banking and government services.
Investigations pointed to compromised Aadhaar enrolment operators and insecure API integrations between government agencies and private firms as the root cause of the breach.
🔹 Impact: Large-scale identity fraud, banking fraud, and reputational damage to government institutions.
🔹 Lesson: Aadhaar-linked biometric data needs advanced encryption, zero-trust frameworks, and continuous cybersecurity monitoring to prevent future leaks.
Strengthening India’s Biometric Security
The cases above illustrate the critical cybersecurity threats facing India’s biometric ecosystem. To mitigate risks, the following measures should be enforced:
✅ Multi-Layer Authentication: Combine biometrics with PINs, passwords, or behavioural authentication.
✅ AI-Powered Fraud Detection: Deploy AI-based monitoring systems to detect abnormal biometric access patterns.
✅ Biometric Encryption & Blockchain Security: Encrypt stored biometric data and use blockchain-based authentication to prevent tampering.
✅ Regulatory Compliance & User Awareness: Strengthen Data Protection Laws and educate users on securing their biometric information.
While biometrics offers enhanced security and convenience, its vulnerabilities pose significant risks. India must invest in robust cybersecurity frameworks, continuous threat monitoring, and regulatory oversight to safeguard its biometric infrastructure from cyber threats.
Emerging Threats to Next-Generation Biometric Systems
1. Adversarial Generative Attacks
AI-driven generative adversarial networks (GANs) can create imperceptible perturbations, deceiving biometric systems in real-time. These attacks can bypass conventional detection methods and compromise authentication.
2. Contextual Spoofing
By exploiting environmental variables like lighting, motion, or sound, attackers degrade the reliability of biometric systems. For instance, inconsistent lighting conditions may trick facial recognition algorithms.
3. Quantum-Assisted Biometric Decryption
Future quantum computing capabilities could break existing encryption protecting stored biometric templates. This poses a long-term risk to the confidentiality and integrity of biometric data.
4. Temporal Identity Drift Exploitation
Behavioural biometrics such as typing patterns or walking gaits evolve over time. Attackers can exploit these subtle variations to mimic authorised users and evade detection.
5. Synthetic Multi-Modal Fusion Attacks
AI can generate artificial biometric identities by combining fake fingerprints, facial patterns, and voice signals. These sophisticated forgeries challenge current detection mechanisms.
6. Edge AI Poisoning
Localised processing on edge devices is vulnerable to poisoning attacks. By altering training data or inference results, adversaries can subtly manipulate authentication outcomes.
7. Continuous System Adversarial Feedback Loops
Attackers can use iterative testing to adapt to evolving learning algorithms, bypassing even self-learning biometric systems.
8. Predictive Biometric Mapping
By analysing biometric patterns over time, AI can predict future changes, allowing pre-emptive manipulation of long-term identification systems.
Strategic Approaches for Mitigating Biometric Threats
1. AI Risk Scoring
Implement real-time risk evaluation frameworks that assess the integrity of each biometric authentication attempt. This can help identify anomalies and flag suspicious activity.
2. Enhanced Encryption Mechanisms
Adopt post-quantum cryptography techniques to future-proof biometric data against quantum-assisted attacks. Secure data both in transit and at rest using advanced encryption protocols.
3. Decentralised Identity Management
Edge ledger systems enable decentralised biometric management, reducing the risk of centralised data breaches. Blockchain-based identity verification adds an additional layer of security.
4. Self-Learning and Adaptive Engines
Deploy autonomous systems that continuously update authentication baselines, making it harder for attackers to predict or manipulate future states.
5. Contextual Multi-Factor Authentication
Combine physical, behavioural, and contextual data from multiple devices to create robust, adaptive authentication protocols.
6. Regular Penetration Testing
Conduct periodic red-team exercises focused on biometric systems to identify vulnerabilities proactively. Simulating adversarial attacks can strengthen system resilience.
7. Edge AI Security Protocols
Enforce strict security policies for edge devices, including secure boot processes and integrity checks. Implementing federated learning helps minimise the risk of poisoned datasets.
Industry Use Cases: Practical Applications of Secure Biometric Systems
- AI-Driven Risk Assessment: Financial institutions utilise biometric systems for real-time identity verification and fraud detection.
- Advanced Payment Systems: Biometric authentication ensures secure, seamless, and user-friendly payment experiences.
- Critical Infrastructure Access Control: Multi-modal biometrics enhance physical security for critical sectors such as power plants and transportation networks.
- Healthcare Identity Management: Biometrics provide secure patient identification and facilitate accurate record-keeping.
- IoT Device Authentication: Biometric gateways ensure only authorised users can interact with IoT devices, reducing unauthorised access risks.
Business Impact and Return on Investment (ROI)
Securing next-generation biometric systems is an investment in both operational integrity and customer trust. Key business benefits include:
- Risk Mitigation: Reducing vulnerabilities safeguards against financial and reputational damage.
- Compliance Assurance: Meeting global regulatory standards (e.g., GDPR, PDP Bill) protects businesses from legal liabilities.
- Customer Confidence: Robust security fosters user trust, enhancing brand loyalty and reducing churn.
- Operational Efficiency: Automated and adaptive systems streamline identity management, cutting manual oversight costs.
- Future-Readiness: Implementing advanced defences ensures resilience against emerging threats, preserving long-term value.
Quantum-Assisted Biometric Decryption: A Looming Threat to Data Security
The rapid advancement of quantum computing presents a transformative potential across various industries, from pharmaceuticals to artificial intelligence. However, this disruptive technology also poses a significant threat to cybersecurity, particularly to biometric data encryption. Future quantum capabilities could dismantle existing cryptographic protocols, endangering the confidentiality and integrity of stored biometric templates. This article explores the risks of quantum-assisted biometric decryption, its implications for businesses and individuals, and the countermeasures required to mitigate this looming cyber threat.
The Quantum Threat to Biometric Security
Biometric authentication—such as fingerprint scans, iris recognition, and facial identification—relies on complex cryptographic techniques to secure biometric templates stored in databases. Currently, these templates are encrypted using classical cryptographic algorithms such as RSA and ECC (Elliptic Curve Cryptography). However, quantum computing, through algorithms like Shor’s algorithm, has the potential to break these encryption methods exponentially faster than classical computers.
When quantum computers reach sufficient computational power, they could decrypt stored biometric data, leading to severe security breaches. Unlike passwords, biometric data is immutable—once compromised, it cannot be reset or changed. This presents a unique challenge in the cybersecurity landscape.
Potential Implications of Quantum-Assisted Biometric Decryption
1. Identity Theft and Fraud
Biometric data serves as a unique identifier, often linked to passports, banking systems, and secure access controls. If quantum-assisted attacks compromise these databases, criminals could impersonate individuals for fraudulent activities, rendering biometric authentication unreliable.
2. Nation-State Espionage
Quantum computing is a strategic asset for many governments, with leading nations investing heavily in quantum research. Adversarial states could leverage quantum decryption techniques to gain access to critical biometric databases, compromising national security and intelligence operations.
3. Corporate Espionage and Data Breaches
Enterprises that rely on biometric access control could become vulnerable to data breaches. Cybercriminals or rival corporations could use quantum-assisted attacks to bypass security measures, leading to financial and reputational damage.
4. Erosion of Public Trust in Biometric Authentication
The widespread use of biometric authentication in smartphones, border control, and financial transactions relies on public trust. If quantum computing undermines biometric security, individuals and organisations may be reluctant to adopt these technologies, leading to operational inefficiencies and increased reliance on less secure authentication methods.
Countermeasures: Preparing for the Quantum Era
To mitigate the risks of quantum-assisted biometric decryption, organisations must adopt proactive measures to future-proof their cybersecurity strategies.
1. Post-Quantum Cryptography (PQC)
The development of quantum-resistant cryptographic algorithms is crucial. The National Institute of Standards and Technology (NIST) is actively working on post-quantum cryptographic standards that will replace vulnerable encryption methods. Biometric security systems must transition to these quantum-resistant encryption techniques to ensure long-term protection.
2. Quantum Key Distribution (QKD)
Quantum key distribution offers a novel approach to secure communications by leveraging the principles of quantum mechanics. QKD ensures that encryption keys remain secure even in a quantum computing era, significantly enhancing biometric data protection.
3. Decentralised and Privacy-Preserving Biometrics
Implementing privacy-preserving biometric techniques, such as homomorphic encryption and decentralised biometric storage, can reduce the risk of centralised data breaches. Instead of storing biometric templates in vulnerable databases, biometric authentication could utilise secure multi-party computation or blockchain technology for added security.
4. Hybrid Cryptographic Systems
A gradual shift to hybrid cryptographic models—integrating both classical and post-quantum encryption—can provide an interim solution before fully transitioning to quantum-resistant algorithms. This approach ensures that biometric data remains secure during the transition period.
5. Regulatory Compliance and International Collaboration
Governments and cybersecurity agencies must establish regulations and frameworks that mandate quantum-resistant encryption for biometric data. Global collaboration between academia, industry, and government entities is essential to accelerate the adoption of quantum-secure technologies.
Penetration Testing Biometrics: Securing the Future of Identity Verification
Biometric authentication, once a futuristic concept, has become a mainstream security measure across industries. From fingerprint scanners on smartphones to facial recognition systems in banking and law enforcement, biometric technologies promise enhanced security and convenience. However, they are not impervious to cyber threats. As organisations increasingly rely on biometrics for identity verification, penetration testing emerges as a critical process to ensure their resilience against sophisticated attacks.
This blog explores penetration testing in biometric security, highlighting its significance, methodologies, real-world threats, and best practices for safeguarding biometric systems.
Why Penetration Testing is Essential for Biometric Systems
Unlike passwords, which can be reset if compromised, biometric data—such as fingerprints, facial features, iris patterns, and voice recognition—are immutable. A breach of biometric databases can have long-term consequences, making security paramount. Penetration testing, or ethical hacking, proactively identifies vulnerabilities in biometric authentication systems before malicious actors can exploit them.
Key reasons to conduct biometric penetration testing include:
- Preventing Spoofing Attacks – Hackers use deepfake technology, synthetic fingerprints, or voice mimicry to bypass biometric security.
- Ensuring Data Integrity – Biometric data stored in databases must be protected from modification, theft, or unauthorised access.
- Mitigating API and Middleware Vulnerabilities – Many biometric systems rely on APIs for integration, which, if insecure, can be exploited.
- Compliance with Regulations – Organisations handling biometric data must adhere to GDPR, CCPA, and other privacy laws, which mandate security measures.
- Strengthening Multi-Factor Authentication (MFA) – Many systems use biometrics alongside passwords or OTPs, requiring robust security testing.
Common Attack Vectors in Biometric Systems
Penetration testers simulate real-world cyberattacks to uncover weaknesses in biometric systems. Below are some prevalent attack techniques:
1. Presentation Attacks (Spoofing)
- Fingerprint Cloning: Attackers use high-resolution images to create synthetic fingerprints.
- Facial Recognition Spoofing: Deepfakes, 3D masks, or printed photos deceive face recognition systems.
- Voice Imitation: AI-generated voice synthesis can trick voice authentication mechanisms.
2. Database and Storage Attacks
- Biometric Data Theft: If stored without encryption, biometric data is susceptible to breaches.
- Template Reconstruction Attacks: Hackers attempt to reverse-engineer stored biometric templates into raw biometric data.
3. Replay and Man-in-the-Middle (MITM) Attacks
- Intercepting Biometric Data: If biometric authentication relies on network transmission, attackers can intercept and replay data to gain access.
- Altering Authentication Requests: By manipulating API calls, attackers can bypass biometric security.
4. Brute-Force and Machine Learning-Based Attacks
- Brute-Force Matching: Exploiting weak biometric matching thresholds to gain unauthorised access.
- AI-Powered Attacks: Machine learning algorithms are trained to generate synthetic biometric traits that bypass security checks.
Penetration Testing Methodologies for Biometrics
A robust penetration testing strategy for biometric systems includes:
1. Reconnaissance and Threat Modelling
- Identifying all biometric entry points (fingerprint, iris, voice, etc.).
- Understanding system architecture, including APIs and databases.
- Mapping potential attack surfaces.
2. Exploiting Physical and Digital Weaknesses
- Presentation attack testing: Using fake fingerprints, masks, and deepfake videos.
- Database security assessment: Identifying weak encryption, unprotected storage, and poor access controls.
- Network penetration testing: Testing for MITM, API vulnerabilities, and unauthorised data interception.
3. Code and System Analysis
- Reverse Engineering: Analysing biometric software for vulnerabilities.
- API Fuzzing: Sending unexpected inputs to test for system crashes or security gaps.
- Security Misconfigurations: Identifying weak default settings and access privileges.
4. Reporting and Remediation
- Documenting findings with risk severity and impact analysis.
- Suggesting remediation steps, such as strengthening encryption, implementing liveness detection, and enhancing API security.
Best Practices for Securing Biometric Systems
- Implement Liveness Detection – Prevent spoofing by verifying real-time biological responses like eye blinking or voice modulation.
- Encrypt Biometric Data – Use strong encryption standards (AES-256, SHA-3) to protect stored biometric information.
- Use Multi-Factor Authentication (MFA) – Combine biometrics with PINs, smart cards, or behavioural analytics.
- Secure APIs and Middleware – Enforce authentication, access controls, and rate-limiting on biometric APIs.
- Regular Penetration Testing – Conduct frequent security assessments to stay ahead of evolving threats.
- Adopt Zero Trust Architecture – Ensure that biometric authentication is continuously validated with strict access policies.
Biometric authentication is transforming digital security, but it is not invulnerable. Penetration testing plays a crucial role in strengthening biometric defences against evolving cyber threats. Organisations must adopt a proactive security approach by testing, identifying vulnerabilities, and implementing robust countermeasures. By doing so, they can ensure the integrity of biometric systems and safeguard user identities in an increasingly digital world.
Final Thoughts
Quantum-assisted biometric decryption is not a distant theoretical threat but an imminent challenge that requires immediate attention. As quantum computing capabilities advance, organisations and governments must invest in post-quantum cryptographic solutions to safeguard biometric data. The transition to quantum-resistant security frameworks will determine the resilience of biometric authentication in the digital age.
Proactive measures, such as post-quantum cryptography, quantum key distribution, and decentralised biometric solutions, will be essential in mitigating the risks associated with quantum-assisted cyber threats. Organisations that act now will not only protect sensitive biometric data but also maintain trust and security in an increasingly digital world.
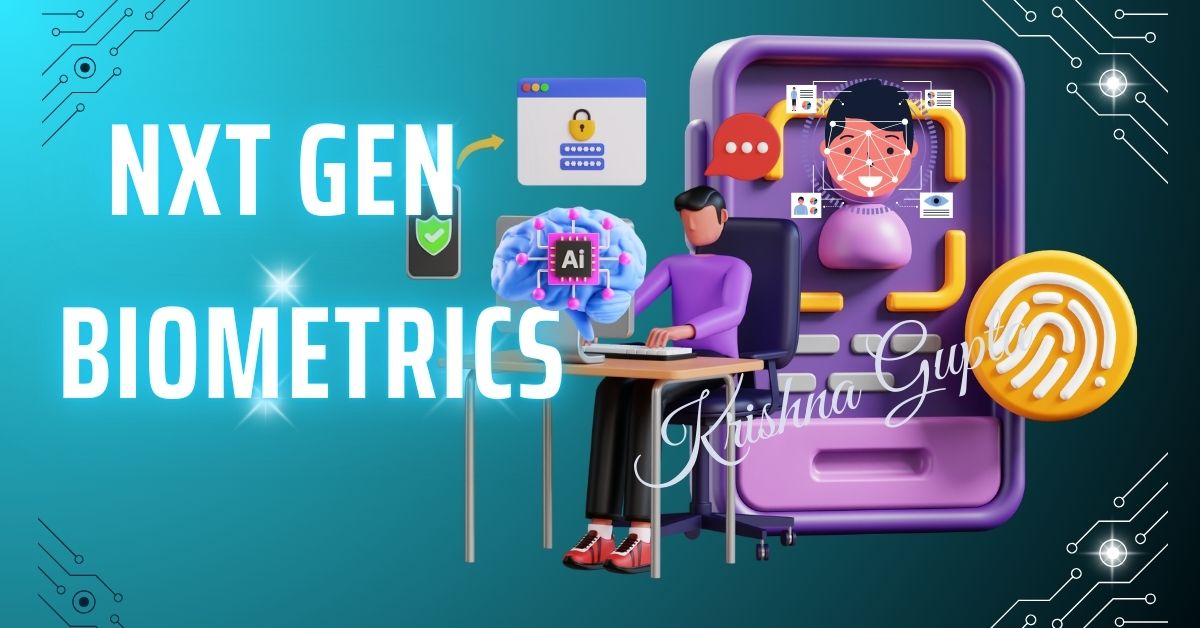
As biometric technologies evolve, so too do the threats they face. By adopting a proactive, multi-layered security strategy, businesses can safeguard sensitive systems against AI-powered attacks. This not only protects critical infrastructure but also enhances customer confidence and operational resilience. In an increasingly digital India, securing next-generation biometric systems is not just a technical challenge—it is a strategic imperative for long-term business success.