Explainable AI (XAI): Building Trust, Transparency, and Tangible ROI in Enterprise AI
Executive Summary
In today’s AI-driven economy, explainability is no longer optional—it is mission-critical. While machine learning and deep neural networks fuel remarkable breakthroughs, their “black-box” nature has become a pressing concern. From regulatory compliance to stakeholder trust, businesses must now prioritise not only what decisions AI makes but also why.
This blog demystifies Explainable AI (XAI) for C-level decision-makers and AI practitioners, shedding light on its business value, technical underpinnings, and strategic importance across sectors. Whether you’re deploying AI in financial modelling, healthcare diagnostics, autonomous systems, or cybersecurity, the ability to interpret, audit, and trust AI decisions has a direct impact on your bottom line and brand reputation.
Table of Contents
- Introduction: Why Explainable AI Matters
- Defining Explainable AI: Beyond the Buzzword
- The Business Case for XAI
- Regulatory and Legal Implications
- Core Techniques in Explainable AI
- Case Studies: Explainability in Action
- Strategic Implementation Roadmap
- XAI in Risk Mitigation and Governance
- Challenges and Limitations
- Future Outlook: From XAI to Trustworthy AI
- Final Thoughts
1. Introduction: Why Explainable AI Matters
As enterprises scale their AI initiatives, the conversation has shifted from “Can we do this?” to “Should we do this—and how can we explain it?”. According to Gartner, by 2026, 60% of large organisations will require AI models to be explainable to ensure responsible decision-making.
In high-stakes domains—healthcare, finance, defence, and law enforcement—opaque models pose significant risks, including bias, ethical lapses, and costly litigation. Explainability is now central to:
- Stakeholder trust
- Regulatory adherence
- Model validation
- Operational resilience
For executives, the call is clear: transparency is a strategic asset.
2. Defining Explainable AI: Beyond the Buzzword
What is Explainable AI?
Explainable AI refers to methods and techniques that make the decision-making processes of AI systems comprehensible to humans. Unlike traditional software with deterministic logic, most AI models learn patterns from data, making their internal workings difficult to understand.
There are two types of explainability:
- Global Explainability: Understanding how the model behaves in general.
- Local Explainability: Explaining individual predictions or decisions.
Key Terms
Term | Definition |
Interpretability | How well a human can understand the cause of a decision |
Transparency | Degree to which model mechanics are visible |
Justifiability | Ability to justify decisions in a human-understandable way |
Auditability | Ability to track, review, and validate AI decisions and data |
3. The Business Case for XAI
ROI and Competitive Advantage
- Improved Decision-Making: Executives gain visibility into model logic, enhancing strategic planning.
- Faster Troubleshooting: Debugging becomes efficient when model predictions are interpretable.
- Reduced Regulatory Fines: Compliance with GDPR, HIPAA, and AI Act mandates explainability.
- Higher Adoption Rates: Stakeholders are more likely to use systems they can understand.
- Brand Trust: Transparency enhances customer loyalty and corporate reputation.
Quantifiable Outcomes
- 40% reduction in model debugging time (McKinsey)
- 20–30% improvement in stakeholder confidence in pilot programs
- 30% less regulatory churn for AI projects in finance and healthcare
4. Regulatory and Legal Implications
Explainability is no longer a technical preference—it’s a legal necessity. Major regulations mandating XAI include:
Regulation | Requirement |
EU AI Act | High-risk AI must be transparent and auditable |
GDPR Article 22 | Right to explanation for automated decisions |
US AI Bill of Rights | Emphasises explainability, privacy, and discrimination safeguards |
India’s DPDP Bill 2023 | Mandates clear accountability for automated processing |
Failing to explain AI decisions can expose businesses to:
- Class-action lawsuits
- Hefty fines (up to 6% of global revenue under EU AI Act)
- Revocation of licences in sensitive industries
5. Core Techniques in Explainable AI
5.1 Model-Specific vs Model-Agnostic Methods
Method Type | Examples | Description |
Model-Specific | Decision Trees, Linear Models | Inherently interpretable by design |
Model-Agnostic | LIME, SHAP, Anchors | Work with any model post-training to offer explanations |
5.2 Popular XAI Tools
Tool/Library | Usage | Best Suited For |
SHAP | Shapley values for feature importance | Tabular data, ensemble models |
LIME | Local Interpretable Model-Agnostic Explanations | Image, text, and tabular predictions |
What-If Tool | Interactive visual tool (by Google) | Fairness and bias testing |
IBM AI360 | Bias detection, explainability | Enterprise governance frameworks |
6. Case Studies: Explainability in Action
6.1 Healthcare – AI in Diagnostics
Use Case: AI model predicting cardiovascular risk.
Without XAI: Doctors rejected the model due to unexplained anomalies.
With SHAP: Cardiologists understood which biomarkers influenced predictions—leading to 32% faster adoption.
6.2 Finance – Loan Approval Systems
Use Case: Credit scoring model for retail loans.
Without XAI: Regulatory pushback due to opaque decisions.
With LIME: Compliance officers could review individual denials—resulting in 12% fewer rejections overturned.
6.3 Cybersecurity – Threat Detection
Use Case: AI flagging anomalous behaviour in corporate networks.
With Explainability Dashboards: Security teams could distinguish between benign anomalies and real threats—cutting false positives by 48%.
7. Strategic Implementation Roadmap
Step 1: Align with Business Use Cases
Prioritise XAI in areas with:
- High compliance requirements
- Stakeholder-facing outputs
- Complex, high-impact decisions
Step 2: Choose the Right Model
If explainability is paramount, prefer interpretable models over deep learning.
Step 3: Integrate XAI Tools
Adopt open-source tools and integrate them into MLOps pipelines.
Step 4: Upskill Teams
Train AI, legal, and business teams on XAI tools and compliance obligations.
Step 5: Monitor Continuously
Use dashboards and alerts to maintain explainability across model drift, retraining, and feedback loops.
8. XAI in Risk Mitigation and Governance
Explainability fortifies risk governance by:
- Detecting bias early: Spot discrimination in training data.
- Reducing operational risk: Explainability prevents flawed automation.
- Enabling forensic audits: Useful in breach investigations or regulatory scrutiny.
- Supporting Incident Response: Helps teams understand how and why a breach occurred.
For CISOs and risk officers, XAI is now a compliance control and incident response aid.
9. Challenges and Limitations
Challenge | Description |
Trade-off with accuracy | Simpler models may explain better but perform worse |
Explanation quality | Not all methods give intuitive insights for laypersons |
Performance overhead | Real-time explainability tools may slow inference |
Lack of standardisation | No universal metrics to quantify “how explainable” a model is |
Overcoming these challenges requires careful model architecture planning and cross-functional collaboration.
10. Future Outlook: From XAI to Trustworthy AI
Explainability is one pillar of Trustworthy AI, alongside:
- Fairness
- Privacy
- Accountability
- Robustness
Emerging trends:
- Causal AI: Explains not just correlations but causal relationships.
- Neuro-symbolic systems: Merge neural networks with logical rules.
- Regulatory sandboxes: Allow AI firms to pilot XAI in real-world environments under supervision.
The trajectory is clear: AI systems that cannot justify their decisions will be relegated to the sidelines.
11. Final Thoughts
Explainable AI is not merely a technical feature—it’s a business imperative, a regulatory safeguard, and a trust-building mechanism. For C-Suite leaders and AI architects, it is the keystone to deploying safe, auditable, and profitable AI systems.
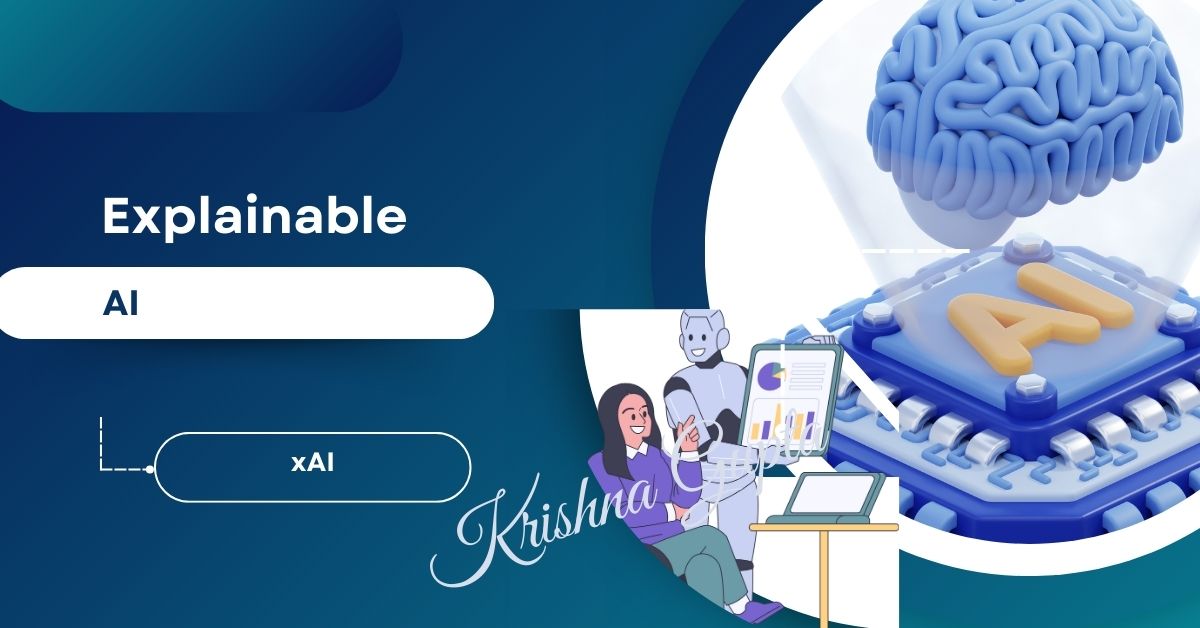
In a world driven by data, explanation is the new validation.