Amazon Redshift: Revolutionising Data Warehousing for C-Suite
In today’s data-driven landscape, organisations face an increasing need to store, analyse, and derive actionable insights from vast datasets. For enterprises relying on the AWS ecosystem, Amazon Redshift emerges as a premier cloud data warehousing solution. Fully managed and seamlessly integrated with other AWS services, Redshift provides the scalability, performance, and analytical depth necessary for modern businesses. This post delves into Amazon Redshift’s features, benefits, and use cases, offering actionable insights tailored for C-Suite executives evaluating its business impact.
Understanding Amazon Redshift
Amazon Redshift is Amazon Web Services’ (AWS) fully managed cloud data warehouse designed for large-scale data analytics. Its architecture facilitates querying petabyte-scale datasets while ensuring cost efficiency and high performance. Built on a massively parallel processing (MPP) framework, it distributes workload across multiple nodes, enhancing speed and reliability.
Redshift’s standout quality is its seamless integration within the AWS ecosystem, allowing organisations to unify their data strategies with tools like Amazon S3, Glue, and EMR.
For C-Suite executives, Redshift’s ability to convert complex datasets into meaningful business insights translates into improved decision-making, operational efficiency, and competitive advantage.
Key Features of Amazon Redshift
1. Highly Scalable and Petabyte-Ready
Amazon Redshift can handle datasets ranging from gigabytes to petabytes, ensuring scalability as businesses grow. Its architecture accommodates the addition of nodes to the cluster seamlessly, allowing organisations to scale up or down based on demand without downtime.
Why It Matters to Executives:
- Scalability ensures business continuity during periods of rapid growth or seasonal spikes.
- Eliminates the need for costly on-premises hardware investments.
- Aligns with cloud-first strategies, reducing total cost of ownership (TCO).
2. Seamless Integration with AWS Services
Amazon Redshift works harmoniously with the broader AWS ecosystem:
- Amazon S3: For storing and querying massive data lakes.
- AWS Glue: For data preparation and ETL (extract, transform, load) workflows.
- Amazon EMR: For distributed data processing.
- QuickSight: For advanced visualisation and reporting.
Executive Takeaway:
By leveraging Redshift’s integrations, organisations can streamline their data pipelines and ensure faster time-to-insight. The unified ecosystem reduces operational friction, enabling focus on strategic goals rather than technical bottlenecks.
3. Machine Learning-Based Query Optimisation
Redshift employs machine learning (ML) to optimise query execution, improving speed and reducing costs. Features like Autovacuum, Result Caching, and Concurrency Scaling ensure minimal latency and peak performance, even during high-query workloads.
Business Implications:
- Faster queries lead to better real-time decision-making.
- Predictable performance under varying workloads mitigates risks associated with downtime or delays.
- ML-driven efficiencies lower operational costs without compromising on output.
Why Amazon Redshift Is Ideal for Organisations Using AWS
For organisations already operating within AWS, Redshift represents a natural extension of their infrastructure. Here’s why:
1. Enhanced ROI through Ecosystem Synergy
AWS users can leverage shared security policies, pricing models, and performance metrics across services. Redshift’s pay-as-you-go pricing model aligns with CFO priorities for financial predictability and cost efficiency.
2. Risk Mitigation with Robust Security
Security is a board-level concern. Redshift incorporates industry-leading measures such as:
- End-to-End Encryption: Data in transit and at rest is encrypted using AWS Key Management Service (KMS).
- Network Isolation: Virtual Private Cloud (VPC) ensures only authorised access.
- Audit Trails: Integration with AWS CloudTrail provides comprehensive monitoring and compliance.
Executive Insight:
By addressing regulatory and compliance concerns, Redshift minimises legal and reputational risks.
Real-World Use Cases
1. Financial Services
A global financial institution used Redshift to optimise fraud detection by analysing transactional data in real time. This reduced response times from hours to seconds, improving customer satisfaction and regulatory compliance.
2. Retail
A multinational retailer employed Redshift to analyse customer purchasing patterns, enabling dynamic inventory management and personalised marketing campaigns.
3. Healthcare
A leading healthcare provider utilised Redshift to centralise patient records, improving care coordination while meeting stringent data privacy standards.
Strategic Considerations for Implementation
1. Data Governance
Before migrating to Redshift, executives should establish clear policies on data ownership, access, and compliance.
2. Cost Optimisation
While Redshift’s on-demand pricing model is appealing, businesses must monitor query patterns and storage usage. Leveraging Reserved Instances can yield significant savings for predictable workloads.
3. Skills Development
To maximise Redshift’s potential, organisations should invest in upskilling their workforce, particularly in SQL and AWS-native tools.
Redshift vs. Competitors
Amazon Redshift competes with other cloud data warehouse solutions like Google BigQuery and Snowflake. While all offer robust capabilities, Redshift’s deep AWS integration and petabyte-scale support make it uniquely suited for AWS-aligned organisations.
Feature | Amazon Redshift | Google BigQuery | Snowflake |
AWS Integration | Best in Class | Moderate | Moderate |
Pricing Model | Flexible (pay-per-query or reserved nodes) | Per-query | Per-storage + compute |
Scalability | Petabyte-scale | High | High |
Machine Learning | Built-in | Limited | Limited |
Why C-Suite Executives Should Prioritise Redshift
Amazon Redshift’s robust features, seamless AWS integration, and scalability make it an invaluable asset for enterprises seeking to harness the power of big data. For C-Suite executives, Redshift represents not just a technological upgrade but a strategic enabler, driving:
- Business Impact: Improved data-driven decision-making.
- Return on Investment (ROI): Cost efficiency through cloud adoption.
- Risk Mitigation: Enhanced security and compliance.
In a rapidly evolving digital ecosystem, investing in solutions like Amazon Redshift positions organisations to remain agile, competitive, and innovative.
Here are several alternatives to Snowflake (a cloud-based data platform) that cater to different business needs, offering options in data warehousing, analytics, and cloud-native solutions:
1. Amazon Redshift
- Overview: Amazon’s fully managed cloud data warehouse solution, integrated with the AWS ecosystem.
- Key Features:
- Highly scalable, with support for petabyte-scale datasets.
- Seamless integration with other AWS services (e.g., S3, EMR, Glue).
- Machine learning-based optimisations for query performance.
- Best For: Organisations already using AWS infrastructure.
2. Google BigQuery
- Overview: A fully managed, serverless data warehouse on the Google Cloud Platform (GCP).
- Key Features:
- Pay-per-query pricing model, offering cost control for large datasets.
- Strong machine learning integration with Vertex AI and TensorFlow.
- Real-time analytics capabilities via Dataflow integration.
- Best For: Data science-heavy teams or businesses invested in the GCP ecosystem.
3. Microsoft Azure Synapse Analytics
- Overview: A cloud-based analytics service integrating big data and data warehousing.
- Key Features:
- Combines on-demand and provisioned resources for flexibility.
- Tight integration with Azure’s ecosystem (Power BI, Azure ML).
- SQL-based analytics with Apache Spark for big data processing.
- Best For: Enterprises using Azure cloud services.
4. Databricks Lakehouse Platform
- Overview: Combines data lakes and warehouses for unified data analytics.
- Key Features:
- Optimised for machine learning and AI workloads.
- Built on Apache Spark for distributed data processing.
- Delta Lake technology ensures data reliability and performance.
- Best For: AI/ML-focused companies needing a unified data platform.
5. Cloudera Data Platform (CDP)
- Overview: A hybrid data platform for analytics across public and private clouds.
- Key Features:
- Open-source foundation with Apache Hadoop and Apache Hive.
- Supports edge-to-AI solutions, including streaming analytics.
- Built for hybrid or multi-cloud environments.
- Best For: Organisations with legacy Hadoop setups or hybrid deployments.
6. Teradata Vantage
- Overview: A multi-cloud data analytics platform for enterprise-grade workloads.
- Key Features:
- Supports hybrid cloud, multi-cloud, and on-premises setups.
- Advanced analytics with integrated AI/ML capabilities.
- Strong enterprise focus with robust security and governance tools.
- Best For: Enterprises with complex data needs requiring robust governance.
7. IBM Db2 Warehouse
- Overview: A cloud-native data warehouse platform by IBM.
- Key Features:
- Built for scalability and AI-driven analytics.
- Integration with IBM Watson and IBM Cloud Pak for Data.
- Strong focus on data security and governance.
- Best For: Businesses leveraging IBM’s ecosystem or hybrid solutions.
8. Oracle Autonomous Data Warehouse
- Overview: Oracle’s fully autonomous data warehouse service.
- Key Features:
- AI-driven for automated tuning, scaling, and patching.
- Integration with Oracle’s suite of enterprise applications.
- Built-in analytics and visualisation tools.
- Best For: Enterprises already using Oracle products.
9. Firebolt
- Overview: A performance-focused cloud data warehouse optimised for low-latency analytics.
- Key Features:
- Columnar storage with aggressive compression for fast querying.
- Multi-cluster compute engine for high concurrency.
- Built for real-time and interactive analytics.
- Best For: Startups or mid-sized businesses needing fast, real-time insights.
10. Vertica
- Overview: A scalable, analytical database for big data processing.
- Key Features:
- Columnar storage for high-performance analytics.
- Supports deployment on-premises, in the cloud, or hybrid.
- Strong integration with BI tools like Tableau and Power BI.
- Best For: Industries requiring deep analytics, such as finance and telecom.
Each alternative has unique strengths and is suited for specific business scenarios. Choosing the right one depends on factors such as your existing infrastructure, scalability requirements, analytics needs, and budget.
Here’s the information presented in a tabular format:
Alternative | Overview | Key Features | Best For |
Amazon Redshift | Fully managed cloud data warehouse by AWS | Scalable to petabyte datasets; Integration with AWS services; Machine learning-based query optimisations | Organisations using AWS infrastructure |
Google BigQuery | Serverless data warehouse on Google Cloud Platform | Pay-per-query model; Real-time analytics; Integration with Vertex AI and TensorFlow | Data science-heavy teams or GCP users |
Azure Synapse Analytics | Analytics service integrating big data and warehousing | Combines on-demand and provisioned resources; Integrates with Power BI and Azure ML; Supports big data with Apache Spark | Azure-based enterprises |
Databricks Lakehouse | Unified platform for data lakes and warehouses | Built on Apache Spark; Delta Lake for data reliability; Optimised for AI/ML workloads | AI/ML-focused organisations |
Cloudera Data Platform | Hybrid platform for public and private cloud analytics | Supports streaming analytics; Open-source foundation (Hadoop, Hive); Designed for hybrid and multi-cloud environments | Legacy Hadoop users or hybrid deployments |
Teradata Vantage | Multi-cloud data analytics platform | Supports hybrid/multi-cloud setups; AI/ML integration; Robust governance tools | Enterprises with complex data governance needs |
IBM Db2 Warehouse | Cloud-native data warehouse by IBM | AI-driven analytics; Integrates with IBM Watson and Cloud Pak; Strong data security and governance | IBM ecosystem users |
Oracle Autonomous DW | Fully autonomous data warehouse service | Automated tuning/scaling; Built-in analytics tools; Tight integration with Oracle enterprise applications | Oracle product users |
Firebolt | Performance-focused cloud data warehouse | Low-latency queries; Columnar storage with compression; Multi-cluster compute engine | Real-time analytics needs |
Vertica | Analytical database for big data | Columnar storage; Supports on-premises, cloud, or hybrid deployments; Integrates with BI tools (e.g., Tableau, Power BI) | Finance, telecom, and deep analytics users |
Penetration Testing Amazon Redshift: Ensuring Robust Security for Data Warehousing
As organisations increasingly rely on Amazon Redshift to manage vast datasets, its security becomes a pivotal concern, particularly for businesses storing sensitive information. While Redshift integrates advanced security features like encryption, network isolation, and auditing, penetration testing (pentesting) offers an additional layer of assurance. Pentesting simulates real-world attack scenarios to uncover vulnerabilities and reinforce the system’s defences.
This article explores the nuances of penetration testing Amazon Redshift, offering actionable insights for C-Suite executives to mitigate risks, safeguard data, and maintain compliance.
Why Security Matters in Amazon Redshift
1. Nature of Data Stored
Redshift often stores critical business data such as financial records, customer information, and proprietary analytics. A breach could lead to:
- Reputational damage.
- Legal repercussions due to non-compliance with GDPR, HIPAA, or other regulations.
- Loss of competitive advantage.
2. Evolving Threat Landscape
Cloud-based environments, while secure by design, are not immune to threats. Misconfigurations, insider threats, and sophisticated cyberattacks pose significant risks.
Can Amazon Redshift Be Penetration Tested?
Yes, Amazon Redshift can be penetration tested, but with some caveats. AWS enforces strict guidelines for security testing to protect its shared infrastructure and other tenants within the AWS ecosystem. Organisations must adhere to AWS’s penetration testing policy, which requires prior approval for certain types of testing activities.
What Is Allowed?
AWS permits testing of your own resources within Redshift, such as:
- User Authentication Mechanisms
- Access Controls
- Database Query Permissions
What Is Prohibited?
Activities that interfere with AWS operations or impact other users are strictly prohibited, including:
- Testing without explicit permission.
- Denial-of-service (DoS) or distributed DoS (DDoS) attacks.
- Exploiting underlying AWS infrastructure.
Common Vulnerabilities in Amazon Redshift
1. Misconfigurations
- Publicly accessible endpoints.
- Overly permissive IAM roles and policies.
- Unencrypted data storage.
2. Weak Authentication Mechanisms
- Weak or reused passwords.
- Lack of multi-factor authentication (MFA) for administrators.
3. Insufficient Access Controls
- Lack of granular permissions for users and applications.
- Unrestricted query access leading to data exposure.
4. SQL Injection Risks
Attackers may exploit vulnerabilities in query handling to inject malicious SQL commands, potentially gaining access to sensitive data.
Approach to Penetration Testing Amazon Redshift
1. Planning and Scoping
Steps for C-Suite Leaders:
- Define Objectives: Identify what you aim to test, such as data confidentiality or user access.
- Obtain Permissions: Seek explicit approval from AWS for any external pentesting efforts.
- Engage Experts: Partner with qualified penetration testers familiar with cloud environments and AWS policies.
2. Vulnerability Assessment
Identify potential weaknesses by evaluating:
- Cluster Configuration: Ensure private subnets and security groups are properly configured.
- IAM Policies: Check for excessive privileges.
- Encryption Standards: Validate encryption for data at rest and in transit.
Tools Commonly Used:
- AWS Trusted Advisor for misconfiguration checks.
- Burp Suite or OWASP ZAP for web application interfaces connected to Redshift.
- SQLMap for testing SQL injection vulnerabilities.
3. Exploitation Simulation
Simulate controlled attacks to understand how Redshift reacts under different threat scenarios:
- Privilege Escalation: Test if lower-privileged accounts can gain unauthorised access.
- Data Exfiltration: Attempt to extract sensitive data to evaluate encryption robustness.
- Authentication Bypass: Test login systems for vulnerabilities.
4. Post-Testing and Reporting
Deliver a comprehensive report highlighting:
- Vulnerabilities identified.
- Exploitation risks.
- Recommendations for mitigation.
For C-Suite:
Focus on business impact and ROI from implementing these recommendations. For instance:
- Investing in stricter access controls prevents potential million-pound losses from data breaches.
- Optimising configurations aligns with compliance standards, avoiding regulatory fines.
Enhancing Amazon Redshift Security Posture
1. Implement Defence-in-Depth Strategies
Layer security measures to minimise attack vectors:
- Enable VPCs and private endpoints to restrict public access.
- Apply AWS WAF (Web Application Firewall) to monitor traffic.
- Use CloudTrail to log and audit actions.
2. Regular Security Audits
Pentesting should not be a one-off activity. Schedule periodic tests, especially after:
- Adding new data sources.
- Scaling clusters.
- Deploying significant updates.
3. Train Employees
Enhance your team’s awareness of cloud security best practices:
- Conduct regular workshops on secure query writing to prevent SQL injection.
- Train administrators to recognise misconfigurations early.
Penetration Testing vs. AWS Shared Responsibility Model
While AWS secures its global infrastructure, the shared responsibility model means you are responsible for securing your Redshift resources. Penetration testing complements this by:
- Identifying blind spots in your implementation.
- Proactively mitigating risks before they materialise into breaches.
Real-World Case Studies
Case Study 1: Financial Institution
A major bank conducted penetration testing on its Redshift cluster to ensure compliance with GDPR. Testing revealed a misconfigured IAM role that allowed unauthorised access to sensitive financial data. The issue was promptly resolved, preventing potential fines.
Case Study 2: E-Commerce Company
An online retailer tested for SQL injection vulnerabilities in its analytics pipeline feeding into Redshift. The penetration test identified a flaw in how user input was sanitised, which was fixed to safeguard customer data.
Final Thoughts: Why Pentesting Redshift Is a Strategic Imperative
For C-Suite executives, penetration testing Amazon Redshift is not merely a technical exercise—it’s a business-critical investment. It ensures the integrity of your organisation’s data, fortifies compliance, and mitigates risks that could jeopardise your brand and bottom line.
Key takeaways:
- Collaborate with certified pentesters to align with AWS guidelines.
- Prioritise regular testing as part of your cybersecurity strategy.
- View penetration testing as a proactive measure to enhance ROI by preventing costly breaches.
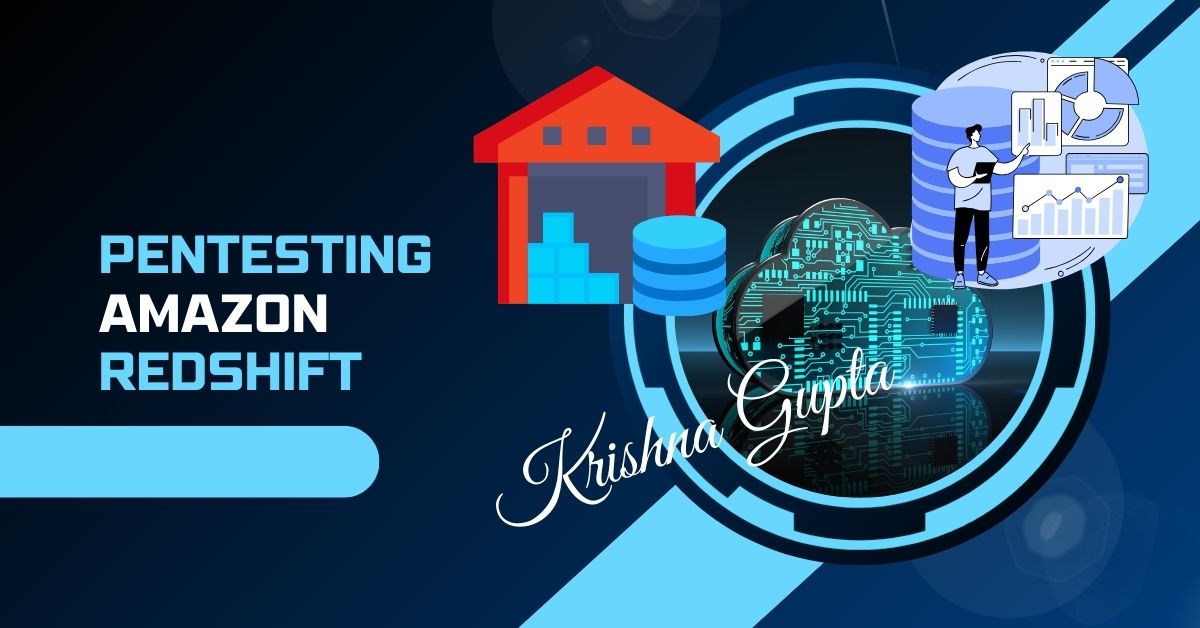
By incorporating penetration testing into your Redshift strategy, your organisation not only fortifies its defences but also demonstrates a commitment to safeguarding stakeholders’ trust.