Agentic AI in Blockchain, Hyperledger, Digital Rupee, and Digital Yuan: A Strategic Guide for C-Suite Executives
Introduction
The convergence of Agentic Artificial Intelligence (AI) with Blockchain technologies, particularly Hyperledger, and digital currencies such as the Digital Rupee and Digital Yuan, is set to redefine the future of enterprise operations, monetary systems, and global economic dynamics. As these technologies evolve, they offer unprecedented opportunities for strategic transformation, but they also carry complex implications that demand careful C-Suite attention.
This in-depth post deciphers the role of Agentic AI in these domains, highlighting strategic insights into ROI, risk mitigation, and operational optimisation for C-Level executives. Whether you are a CEO, CIO, CTO, CFO, or CISO, understanding these developments is essential for informed leadership in the digital economy.
Understanding Agentic AI: More Than Just Autonomous Machines
Agentic AI refers to AI systems that exhibit autonomy, adaptability, and goal-oriented behaviour, making decisions independently and learning dynamically from interactions and outcomes. Unlike traditional AI models that operate within rigid, rule-based boundaries, agentic systems can initiate actions proactively to achieve predefined objectives ā an essential trait for automating complex workflows across digital ecosystems.
Key Characteristics of Agentic AI:
- Goal-directed reasoning
- Autonomous decision-making
- Contextual adaptability
- Multi-agent coordination
- Continuous learning and evolution
In the context of blockchain, digital currencies, and enterprise consortia, agentic AI can act as an intelligent liaison, enforcing rules, verifying transactions, optimising consensus algorithms, and responding in real-time to market dynamics or regulatory changes.
Blockchain and Hyperledger: A Natural Foundation for Agentic AI
Blockchain as a Trust Layer
Blockchain’s decentralised, immutable ledger forms a reliable backbone for autonomous agents. Smart contracts, distributed consensus mechanisms, and cryptographic proof provide the perfect environment for agentic AI to operate transparently and securely.
Agentic AI embedded in blockchain systems can:
- Monitor and verify transactions autonomously
- Detect anomalies or fraud using behavioural patterns
- Interact with smart contracts to enforce compliance
- Make decisions based on immutable historical data
Why Hyperledger?
Hyperledger, hosted by The Linux Foundation, is a set of open-source blockchains and related tools tailored for enterprise use. Unlike public blockchains like Bitcoin or Ethereum, Hyperledger Fabric, Sawtooth, and Besu offer permissioned networks, modular architecture, and fine-grained control over data accessākey requirements for enterprise adoption.
Agentic AI integrated into Hyperledger can:
- Perform dynamic access control decisions
- Optimise chaincode (smart contract) execution
- Trigger automated supply chain workflows
- Act as oracles interfacing with external data streams
Use Case: Supply Chain Management
In a multinational supply chain, an AI agent within Hyperledger could:
- Predict demand fluctuations
- Reallocate inventory
- Negotiate logistics costs
- Trigger smart contract settlements upon delivery confirmation
The Digital Rupee and Digital Yuan: Programmable Money Meets Agentic Intelligence
Central Bank Digital Currencies (CBDCs): The Basics
CBDCs are digital forms of sovereign currency, issued and regulated by central banks. Unlike cryptocurrencies, they are centralised and governed by national authorities, aiming to digitise fiat currency without the volatility of crypto-assets.
Indiaās Digital Rupee (eā¹)
Issued by the Reserve Bank of India (RBI), the Digital Rupee is being rolled out in phases for wholesale and retail use. It is poised to:
- Enhance payment efficiency
- Reduce cash handling costs
- Enable programmable financial instruments
Chinaās Digital Yuan (e-CNY)
The Peopleās Bank of China (PBOC) leads global CBDC adoption through extensive pilot programmes. The Digital Yuan features:
- Controlled anonymity
- Traceable transactions
- Cross-border interoperability (in pilot with Hong Kong, UAE, Thailand)
Where Agentic AI Fits In
Agentic AI enhances CBDC ecosystems in the following ways:
Function | AI Application Example |
Regulatory Compliance | Automatically flag suspicious transactions for anti-money laundering (AML) checks |
Monetary Policy Tools | Analyse macroeconomic indicators to recommend interest rate changes |
Programmable Transactions | Enable conditional disbursements based on AI-defined logic |
Fraud Detection | Monitor behavioural biometrics and transaction patterns |
Cross-Border Settlements | Dynamically select optimal FX corridors based on real-time market data |
Real-world Scenario:
A cross-border remittance from India to China could be initiated by an enterprise AI agent. It would:
- Verify sender credentials
- Check compliance with RBI and PBOC regulations
- Select the best exchange route
- Initiate and monitor settlement on a Hyperledger-based corridor
- Record all transactions immutably for audit trails
Benefits of Agentic AI for C-Suite Leaders
1. Operational Efficiency
AI agents eliminate human bottlenecks in high-frequency decision-making environments, such as FX trades or inventory reallocation. The result? Lower operational costs and enhanced scalability.
2. Real-Time Risk Mitigation
With the ability to monitor transactions and system behaviours in real time, agentic AI systems can detect and respond to anomalies before they escalate.
Example: An AI agent in a banking system spots an unusual transaction sequence. It halts the transaction, escalates it to compliance, and logs the incident for forensic review.
3. Improved Regulatory Compliance
Agentic AI can keep up with evolving regulations by dynamically adapting smart contract rules or modifying transaction pathways ā ensuring ongoing compliance without human intervention.
4. Enhanced Customer Experience
AI agents can automate customer-facing services like KYC onboarding, dispute resolution, or intelligent routing of digital payments, leading to faster and more accurate service delivery.
5. Strategic Insights and Decision Support
By leveraging predictive analytics and simulation capabilities, agentic AI provides the C-Suite with scenario-based planning, strategic forecasting, and competitor benchmarking.
Challenges and Risk Factors
1. Algorithmic Bias
Decisions made by AI agents must be transparent and auditable. C-Suite leaders must ensure their systems are free from bias, especially in lending or hiring decisions.
2. Data Privacy and Governance
While AI thrives on data, privacy laws like GDPR and Indiaās Digital Personal Data Protection Act necessitate strict controls. Blockchainās immutability could conflict with data erasure rights.
3. Interoperability and Legacy Integration
Integrating agentic AI into existing ERP or legacy banking systems can be challenging. Cross-compatibility between blockchain layers (public and permissioned) remains complex.
4. Cybersecurity Threats
Agentic AI introduces a new attack surface. Malicious manipulation of AI agents or adversarial attacks can have cascading effects. Strong encryption, access control, and penetration testing are vital.
5. Talent and Cultural Gaps
Cultural resistance to automation and a shortage of skilled AI/blockchain professionals can hinder adoption. C-Suite must lead organisational change from the top.
Practical Steps for Implementation
1. Begin with Low-Risk, High-Impact Use Cases
Focus on sectors like:
- Trade finance
- Logistics and supply chains
- Digital payment settlements
- Regulatory reporting
2. Partner with Trusted Providers
Engage vendors with proven expertise in agentic AI and blockchain. Hyperledger-certified partners and CBDC pilot participants are a good starting point.
3. Build Governance into Architecture
Establish AI ethics boards, blockchain governance committees, and audit protocols that evolve with the technology.
4. Invest in Cross-Training Talent
Upskill your compliance, finance, and tech teams to understand the interplay between AI, blockchain, and digital currencies.
5. Monitor Global Standards
Track developments by the BIS Innovation Hub, IMF, and ISO on AI governance, CBDC frameworks, and cross-border blockchain standards.
The Future: Autonomous Enterprises Powered by AI Agents
Imagine a future where:
- An AI agent handles your cross-border treasury operations.
- Another agent automatically allocates idle working capital to CBDC staking instruments.
- A third monitors supply chain carbon credits, ensuring ESG compliance and unlocking green incentives.
These are not hypothetical scenarios but emerging realities being tested by central banks, multinationals, and governments.
š Smart Contracts and Agentic AI: A Strategic Intersection
What Are Smart Contracts?
Smart contracts are self-executing agreements with the contract terms directly written into code. They reside on a blockchain, ensuring transparency, immutability, and automation. Once predefined conditions are met, the contract enforces itselfāwithout the need for intermediaries.
Example:
In a supply chain context, a smart contract might automatically release payment once goods are confirmed delivered via GPS data.
What Is Agentic AI?
Agentic AI refers to AI systems that behave like autonomous agents: they can sense their environment, make decisions, pursue goals, and learn from interactions. Unlike traditional AI, theyāre proactive rather than reactive.
Now, imagine combining these smart agents with self-executing smart contracts.
š¤ How They Work Together
1. Dynamic Execution of Smart Contracts
Traditional smart contracts are staticārules are coded once and seldom adapt. With Agentic AI, contracts become dynamic and context-aware.
For instance:
- An AI agent can monitor external data feeds (like weather or market conditions).
- Based on real-time analysis, it can decide whether to execute, delay, or renegotiate a smart contract clause.
- The AI might even initiate a new contract if conditions evolve significantly.
š§ Example: In an agri-tech insurance model, an AI agent could analyse rainfall data in real-time. If a drought is detected, it automatically triggers a payout via a smart contractāwith no human intervention.
2. Multi-Agent Negotiation
Smart contracts combined with Agentic AI unlock automated negotiation and decision-making across multiple agents.
- In complex B2B transactions, AI agents from both parties can negotiate pricing, delivery schedules, or warranties.
- Once terms are agreed upon, the smart contract executes them autonomously.
šÆ C-Suite Value: This reduces negotiation cycles from weeks to minutesācutting down legal and administrative costs dramatically.
3. Compliance and Risk Mitigation
Smart contracts with embedded AI agents can dynamically adapt to changing regulations.
- If a regulation changes, an agent can update the execution logic or flag non-compliance risks.
- In high-stakes industries like finance or healthcare, this can prevent fines and reputational damage.
š Example: A financial institution uses agentic AI to constantly review AML compliance. If flagged, it modifies contract flows or freezes transactions autonomouslyāwithout waiting for manual audits.
4. Decentralised Decision-Making
In decentralised environments like DAO ecosystems or blockchain consortia, Agentic AI enables:
- Autonomous governance
- Distributed trust
- Self-correcting systems
Smart contracts act as the legal foundation; AI agents act as the decision-makers.
š§© Use Case Snapshots
Use Case | Agentic AI Role | Smart Contract Role |
Trade Finance | Analyse invoice legitimacy & credit risk | Release payment upon verified conditions |
Healthcare Data Sharing | Validate patient consent & data utility | Allow access only when criteria met |
Cross-Border Payments | Optimise FX rates, flag sanctions risk | Execute transaction on approval |
Renewable Energy Trading | Predict supply/demand from IoT sensors | Settle energy credits autonomously |
š§ Strategic Takeaway for the C-Suite
- ROI: Reduces transaction latency and manual overhead, improving operational efficiency.
- Risk: Real-time compliance and anomaly detection reduce regulatory and fraud risks.
- Agility: Supports adaptive, scalable, cross-border operations without bottlenecks.
A Strategic Imperative for the C-Suite
The convergence of Agentic AI, Blockchain (Hyperledger), and CBDCs like the Digital Rupee and Digital Yuan is not merely a technological innovationāitās a strategic inflection point. For C-Suite leaders, the imperative is clear: lead with foresight, invest with intention, and govern with accountability.
Those who embrace this trifecta early will not only optimise operations but also redefine competitive advantage for the digital-first economy.
Actionable Takeaways
- CEOs: Drive digital transformation by aligning AI/blockchain with core business strategies.
- CFOs: Explore CBDCs and smart contracts for cost savings and capital efficiency.
- CIOs/CTOs: Architect secure, scalable systems integrating agentic AI into blockchain workflows.
- CISOs: Develop AI-specific threat models and blockchain-secured infrastructure.
- CMOs/COOs: Use AI agents for customer personalisation, order fulfilment, and payment processing.
Letās unpack Penetration Testing (Pen Testing) for Agentic AI ā a crucial yet emerging domain for C-Suite leaders to understand.
š”ļø Penetration Testing Agentic AI: Proactively Securing Autonomous Intelligence
As Agentic AI systems become more autonomous and integrated with critical digital infrastructure (like blockchain, smart contracts, and CBDCs), security testing becomes non-negotiable. Penetration testing is one of the most strategic tools to identify vulnerabilities before adversaries do.
š¤ Why Is Agentic AI Different from Traditional Systems?
Unlike static systems, Agentic AI:
- Makes decisions autonomously
- Interacts with real-world data (via APIs, IoT, etc.)
- Can initiate chain reactions (e.g., activating smart contracts, transferring assets)
- Learns and evolves (especially in multi-agent systems)
Traditional pen testing models need to be reimagined to account for this dynamic behaviour.
š Key Threat Vectors in Agentic AI
Threat Vector | Description | Risk to Business |
Prompt Injection | Manipulating input to hijack AI behaviour | Misconduct, bad decisions, compliance failure |
Model Manipulation | Poisoning the training data or logic | Skewed decision-making, financial loss |
Environment Spoofing | Feeding fake data to the agent | Triggers false actions (e.g., fund transfers) |
Autonomy Exploitation | Exploiting decision loops to cause cascades | Systemic failure, data breach, DDoS |
Smart Contract Integration Bugs | Faulty logic in the AI-contract interface | Unintended contract execution, asset loss |
š§ How to Pen Test Agentic AI: Key Phases
1. Threat Modelling the Agent
- Map out the agent’s perceptionādecisionāaction loop.
- Identify data sources (APIs, IoT, Web3).
- Understand triggers: What makes the AI act?
C-Suite Insight: This step aligns with enterprise risk management. If AI is making decisions on asset movement or compliance checks, thatās board-level risk.
2. Simulating Adversarial Environments
- Use adversarial inputs (e.g., fake weather data for an AI that controls logistics).
- Evaluate how the AI interprets malicious or ambiguous information.
- Check whether it maintains secure behaviour boundaries.
š§ Example: If Agentic AI manages a CBDC wallet and it receives conflicting regulatory updates, does it freeze, act, or escalate?
3. Fuzz Testing AIāSmart Contract Interfaces
- Fuzz all agent inputs that could trigger a smart contract execution.
- Test for:
- Race conditions
- Logic bugs
- Data overflow
- Double-spending exploits (especially with CBDCs)
4. Red Team Simulation with Human-in-the-Loop
Agentic AI is partially autonomous, so involve red teams that simulate:
- Phishing the agent via spoofed comms
- Compromising APIs or sensors it relies on
- Triggering economic logic bombs (e.g., pump-and-dump signals)
š Real-World Tip: In one DAO simulation, red teams used subtle price signal manipulation to trick an AI trader into repeatedly activating loss-making contracts. The losses were automated and significant before human intervention.
š§ Strategic Risk Categories
Risk Type | Description | Pen Test Focus |
Operational Risk | AI misbehaves or acts erratically | Stress tests, input poisoning |
Compliance Risk | Violates laws or misinterprets rules | Regulatory fuzzing, governance testing |
Financial Risk | Automated loss-making decisions | Market simulation, logic loop testing |
Reputational Risk | Public AI failure (e.g., on-chain) | Attack simulations, adversarial PR |
š C-Suite-Level ROI of Pen Testing Agentic AI
Benefit | Strategic Impact |
Prevention of cascade failures | One bad AI decision could activate a series of smart contracts or payments |
Assurance for regulators | Demonstrates proactive risk governance |
Trust for stakeholders | Confidence in AI autonomy and blockchain transparency |
Insurance leverage | Lowers cyber insurance premiums through demonstrable controls |
š§© Agentic AI + Blockchain + CBDCs = A Triple Threat
Pen testing becomes mission-critical when your Agentic AI is:
- Interacting with programmable currencies (Digital Rupee, Digital Yuan)
- Orchestrating hyperledger smart contracts
- Making on-chain decisions in DAOs or DeFi systems
Any flaw can trigger:
- Loss of digital assets
- Regulatory sanctions
- Reputational disaster
š ļø Tools & Frameworks for Pen Testing Agentic AI
- Adversarial Robustness Toolbox (IBM)
- SecML (for model testing)
- OWASP Top 10 for LLMs & AI
- Hyperledger Caliper (for blockchain performance testing)
- MythX, Slither, or Manticore (for smart contract security)
š§ Executive Summary
For C-Suite leaders, penetration testing Agentic AI is not just a technical exerciseāitās a board-level strategy to:
- Prevent cascading AI-driven failures
- Safeguard blockchain-integrated financial systems
- Maintain confidence in CBDC deployments and smart contract automation
Proactive security is cheaper than reactive damage control.
š§Ŗ Real-World Case Studies: Agentic AI, Blockchain, and Smart Contract Security
ā ļø Case Study 1: The DAO Hack (2016) ā Smart Contract Exploit, No Agentic AI
Context:
The DAO (Decentralised Autonomous Organisation) on Ethereum was one of the first large-scale experiments in autonomous smart contracts.
The Flaw:
The smart contract had a reentrancy bug ā a flaw that allowed attackers to recursively call the withdrawal function, draining funds before the balance was updated.
Result:
- Over $60 million USD in Ether was siphoned.
- Ethereum had to hard fork to recover the funds.
- Massive reputational damage to the concept of autonomous decentralisation.
Agentic AI Angle:
Had an Agentic AI system been embedded with real-time transaction monitoring and anomaly detection, it could have identified the non-human transaction patterns, paused the contract, or raised an alert.
C-Suite Takeaway:
Autonomous systems require autonomous guards ā integrating AI agents to monitor smart contract execution adds an adaptive security layer.
š Case Study 2: OpenAI Codex Misuse in Smart Contract Deployment (Theoretical Risk)
Context:
Developers increasingly use AI tools like Codex/GitHub Copilot to auto-generate smart contracts.
The Issue:
A team unknowingly used AI-generated code that missed access control modifiers, leading to a smart contract that could be triggered by anyone.
Outcome:
- The contract was exploited within 24 hours post-deployment.
- $100,000+ in DeFi tokens lost.
Agentic AI Flaw:
An AI assistant trained on bad code patterns created insecure logic. No penetration testing was performed on the AI-generated contracts.
C-Suite Takeaway:
AI accelerating code development must be balanced with rigorous red-teaming. Penetration testing of both AI outputs and integrations is non-negotiable.
š¦ Case Study 3: Digital Yuan Pilots in China ā Controlled AI Automation
Context:
Chinaās Digital Yuan (e-CNY) has integrated smart contracts in pilot phases for:
- Subsidy disbursement
- Supply chain financing
- Cross-border e-commerce
Security Controls:
- Extensive sandbox testing
- Limited agentic AI used for fraud detection, not fund disbursement
- Human-in-the-loop (HITL) model still enforced for high-value transactions
Agentic AI Role:
AI models were deployed to detect fraud and suspicious transaction chains but were not authorised to execute smart contract transactions independently.
Result:
- No major security breach reported to date
- High level of auditability and traceability
- Reinforced Chinaās position as a leader in programmable CBDCs
C-Suite Takeaway:
Carefully calibrated AI autonomy, with strict limits and multi-layered testing, ensures that innovation doesnāt outpace governance.
šø Case Study 4: MakerDAOās Black Thursday (2020) ā DeFi + Market Shock
Context:
MakerDAO is a decentralised lending platform using AI-assisted oracles and smart contracts. On āBlack Thursday,ā ETH crashed 30% in hours.
What Went Wrong:
- Oracle price feeds lagged
- AI-based risk management tools didnāt respond fast enough
- Smart contracts began liquidating collateral for near-zero bids
Financial Losses:
- Millions of dollars in undercollateralised loans
- Borrowers wiped out
- Community backlash
Pen Test Gap: There was no simulation of flash crash + oracle failure + AI delay ā a complex but plausible risk scenario.
C-Suite Takeaway:
Penetration testing must extend to complex event simulations involving AI + blockchain interplay. Adversarial scenario modelling is critical.
š§ Case Study 5: AI Trader Exploit in a DAO Experiment
Context:
An experimental DAO used an Agentic AI to autonomously trade DeFi tokens based on sentiment signals and market data.
Exploit:
- A red team simulated coordinated Twitter sentiment manipulation (buy signals)
- The AI agent reacted as trained ā buying low-liquidity tokens in bulk
- The attacker front-ran the trades and dumped tokens on the AI-led DAO
Impact:
- The DAO lost over $400K in ETH equivalent
- No contract was hacked ā the AIās logic was manipulated
C-Suite Takeaway:
Penetration testing should cover AI cognition and input trustworthiness, not just system integrity. AI can be manipulated without touching the code.
š§© Key Lessons Across Case Studies
Theme | Insight | C-Suite Action |
AI Can Be Fooled | Agentic AI can misinterpret false data or sentiment | Test for adversarial inputs, spoofing |
Code Is Not Enough | Secure code doesnāt equal secure behaviour | Simulate cascading decisions |
Humans Still Matter | Human-in-the-loop saved CBDC pilots | Maintain override and audit layers |
Pen Testing Scope Must Evolve | Testing must simulate AI-driven actions | Involve red-teams, fuzzers, adversarial ML experts |
Decentralisation Magnifies Impact | One AI-agentās bad decision may be irreversible on-chain | Build fail-safes, off-chain validation mechanisms |
šÆ Strategic Recommendations for C-Level Executives
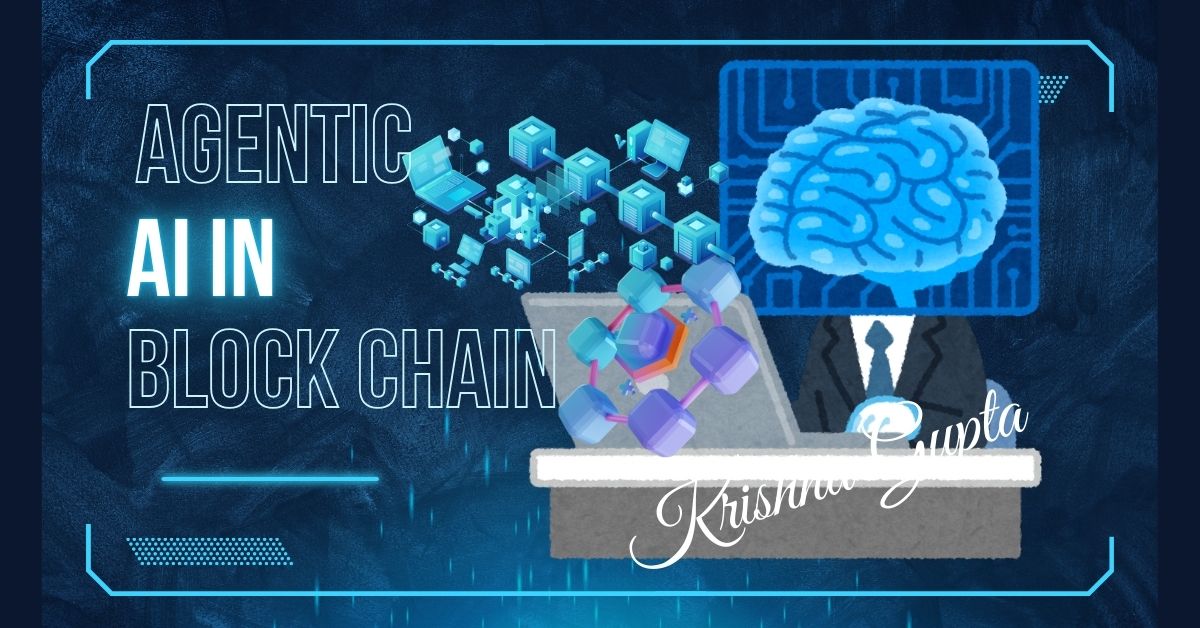
- Mandate Penetration Testing as Part of AIāBlockchain Integrations
ā Especially for smart contracts triggered by autonomous agents. - Invest in Adversarial AI Testing Capabilities
ā Build internal red teams trained in spoofing, manipulation, and economic warfare simulations. - Incentivise Secure AI Development
ā Launch internal bounty programs or red team challenges for AI-agent behaviours. - Adopt Zero-Trust AI Policies
ā Assume agents can be manipulated; enforce external validation checkpoints. - Prioritise Governance in Agentic Systems
ā Include audit trails, override triggers, and stakeholder visibility in AI logic.