AI Agents: The Future of Executive Enablement and Enterprise Intelligence
In the relentless pursuit of operational excellence, strategic foresight, and competitive advantage, Artificial Intelligence (AI) agents are becoming indispensable assets for the modern C-suite. These intelligent digital entities are not merely another technological trend, but powerful instruments capable of reshaping the fabric of enterprise decision-making and performance.
This comprehensive blog post delves deep into the world of AI agents, analysing their nature, capabilities, strategic implications, and potential pitfalls. It is designed specifically for C-level executives seeking to harness cutting-edge innovation to drive value, streamline operations, and future-proof their organisations.
Understanding AI Agents: Definition and Distinction
AI agents are autonomous or semi-autonomous software programmes that perceive their environment, make decisions, and take actions to achieve defined goals. Unlike traditional software, which follows static instructions, AI agents adapt, learn, and optimise over time.
Types of AI Agents
- Reactive Agents – Operate on stimulus-response models without memory.
- Model-Based Agents – Maintain an internal model of the world for decision-making.
- Goal-Based Agents – Make decisions by evaluating future outcomes.
- Utility-Based Agents – Choose actions based on a quantifiable utility function.
- Learning Agents – Improve performance through experience.
These types may be combined in enterprise scenarios, leading to hybrid AI agents tailored for complex, dynamic environments.
The History of AI Agents: From Theory to Enterprise Innovation
AI agents—those autonomous or semi-autonomous systems that perceive, decide, and act—have a history as rich and layered as the broader field of Artificial Intelligence (AI) itself. Understanding this evolution provides context for their growing strategic relevance in today’s business landscape.
1. Origins in Classical AI (1950s–1970s)
The concept of intelligent agents can trace its intellectual roots to the early days of AI research.
- Alan Turing’s 1950 paper “Computing Machinery and Intelligence” laid the philosophical foundation, asking: “Can machines think?”
- The term “agent” wasn’t formalised, but early systems like the Logic Theorist (1956) and General Problem Solver (1957) simulated aspects of human reasoning—rudimentary forerunners to agent-based logic.
- These early AI models were rule-based and lacked adaptability, yet they seeded the notion of machines acting independently within defined parameters.
2. Symbolic and Expert Systems (1970s–1980s)
During this era, expert systems emerged as the first practical implementations of AI-like behaviour in enterprises.
- Systems like MYCIN (medical diagnosis) and XCON (DEC’s configuration expert system) operated within strict domains, using encoded human knowledge.
- These were not agents in the modern sense, as they didn’t learn or adapt dynamically. However, they paved the way for goal-oriented and domain-specific AI applications in business.
3. The Rise of Agent-Based Modelling (1990s)
The 1990s saw the birth of the term “intelligent agent” in mainstream computer science.
- Researchers began modelling autonomous agents capable of interacting with environments and other agents—popular in economic simulations, supply chains, and social behaviour modelling.
- Bradshaw, Jennings, and Wooldridge published foundational work on agent-oriented software engineering and multi-agent systems (MAS).
- Key characteristics emerged: autonomy, social ability, reactivity, and proactivity.
Enterprise adoption was limited during this phase, mostly confined to academic or military simulations.
4. AI Agents in Business Software (2000s–2010s)
As enterprise software matured, AI agents slowly entered the commercial landscape—quietly embedded in tools like:
- Virtual assistants (e.g. early iterations of Siri, Cortana)
- Chatbots and customer service agents
- RPA (Robotic Process Automation) bots with rudimentary decision-making
- Recommendation engines powered by agent-based algorithms (notably in retail and media)
During this time, AI agents gained traction for automating low-level cognitive tasks, often augmenting human workers rather than replacing them.
5. Machine Learning & Deep Learning Revolution (2010s–Present)
Breakthroughs in deep learning, natural language processing (NLP), and big data supercharged the capabilities of AI agents.
- Agents could now learn from experience, recognise images and speech, and respond conversationally.
- Platforms like OpenAI, Google DeepMind, and IBM Watson drove public and enterprise awareness.
- AI agents became cloud-native, API-accessible, and increasingly composable—integrated into enterprise systems, from finance to HR to cybersecurity.
This phase also saw the emergence of conversational agents, digital twins, and AI-driven decision support systems.
6. Generative and Autonomous AI Agents (2020s–Future)
We are now witnessing the rise of generative AI agents and autonomous enterprise agents, capable of:
- Multi-modal input processing (text, images, video, code)
- Handling complex workflows across departments
- Interfacing with APIs and triggering actions independently
- Collaborating in multi-agent ecosystems, performing tasks traditionally reserved for teams of knowledge workers
Tools like AutoGPT, ChatGPT agents, LangChain, and enterprise-specific AI orchestration platforms have introduced memory, tool usage, and self-improvement capabilities—pushing AI agents into strategic, decision-making domains.
What This Means for the C-Suite
Understanding this history is not just an academic exercise—it provides clarity on what’s possible today and what’s coming next:
- Earlier generations of AI agents laid the groundwork with deterministic logic and rule-based systems.
- Modern agents are dynamic, data-driven, and capable of real-time adaptability.
- The future points to autonomous business units, where AI agents negotiate contracts, optimise operations, and drive strategy—with humans in a supervisory role.
The journey of AI agents reflects a shift from automation to augmentation to autonomy. C-level leaders must stay informed and proactive—because those who understand where AI agents have been are best positioned to lead where they’re going.
Would you like me to turn this into a visual timeline or executive briefing slide?
Strategic Relevance of AI Agents in the C-Suite Agenda
AI agents are not simply technical tools—they are strategic enablers. Their growing role in the C-suite’s operational and strategic landscape is a testament to their capacity for high-impact results:
1. Decision Intelligence at Scale
Executives are bombarded with information. AI agents help filter, analyse, and prioritise data, enabling faster and better-informed decisions. With natural language interfaces and integration with enterprise data lakes, agents can provide real-time recommendations, scenario analysis, and predictive insights.
Example: A CFO uses an AI agent to model cash flow under different economic scenarios, adjusting supply chain and pricing strategies accordingly.
2. Operational Efficiency
AI agents streamline workflows by automating repetitive tasks, coordinating across departments, and proactively identifying process bottlenecks.
Example: A COO deploys AI agents to monitor production quality and supply chain KPIs, enabling just-in-time alerts and interventions.
3. Hyper-Personalised Customer Engagement
CMOs are leveraging AI agents to deliver hyper-personalised content, offers, and experiences across channels—based on real-time behavioural data.
Example: An AI agent crafts unique product recommendations and outreach messages for every customer segment, improving conversion rates by 23%.
4. Cybersecurity and Compliance Monitoring
CISOs deploy AI agents to scan networks for threats, detect anomalies, and ensure regulatory compliance in real time.
Example: An AI agent halts an emerging data exfiltration attempt and auto-generates a breach report for audit teams.
5. Board-Level Insight Generation
AI agents summarise and visualise key performance indicators, strategic risks, and external market movements in formats tailored for board presentations.
Example: A CEO receives a daily brief from an AI agent highlighting competitor M&A activity, macroeconomic shifts, and reputational risks.
Real-World Applications and Enterprise Case Studies
Case Study 1: AI Agents in Financial Services
A leading UK bank implemented AI agents to monitor and adjust its lending strategies. By continuously analysing macroeconomic data, borrower behaviour, and repayment patterns, the agents dynamically recalibrated credit models, reducing default rates by 18%.
Case Study 2: Smart Manufacturing
A multinational electronics manufacturer deployed AI agents to manage its global inventory and logistics. The agents predicted material shortages weeks in advance, enabling proactive sourcing and reducing lead times by 27%.
Case Study 3: AI-Enhanced M&A Due Diligence
During a high-stakes acquisition, an AI agent reviewed thousands of legal and financial documents, flagging inconsistencies and anomalies. It saved over 2,000 analyst hours and identified a critical compliance risk, ultimately altering the deal’s structure.
Benefits for the C-Suite: ROI, Risk Mitigation, and Strategic Agility
Return on Investment (ROI)
AI agents offer exponential returns when integrated thoughtfully. Their ability to process vast datasets, identify patterns, and execute tasks leads to tangible cost savings, improved decision-making, and new revenue streams.
Risk Mitigation
By continuously monitoring internal and external signals, AI agents help identify emerging threats early—whether financial, operational, reputational, or cybersecurity-related.
Strategic Agility
With real-time situational awareness and adaptive learning, AI agents empower leaders to respond swiftly to changing market conditions, regulatory shifts, and competitive moves.
Building an AI Agent Ecosystem: What C-Suite Executives Must Know
1. Data Strategy is Foundational
AI agents thrive on data. C-level leaders must ensure high-quality, well-governed, and accessible data repositories across departments.
2. Interoperability and Integration
AI agents must seamlessly integrate with legacy systems, APIs, ERP platforms, and emerging technologies. This requires robust IT architecture and a clear roadmap.
3. Human-AI Collaboration
AI agents are augmenters—not replacements. Culture change, employee enablement, and ethical frameworks are essential for human-AI collaboration.
4. Governance and Transparency
Executives must insist on explainability, auditability, and ethical safeguards in AI agent behaviour. This ensures trust among stakeholders and compliance with evolving AI regulations.
Challenges and Considerations
1. Bias and Ethical Risk
AI agents can perpetuate or amplify biases if trained on unbalanced data. Executives must invest in fairness testing, diverse datasets, and algorithmic transparency.
2. Security Vulnerabilities
AI agents, particularly those operating autonomously, can be exploited. Secure development lifecycles and real-time monitoring are non-negotiable.
3. Over-Reliance and De-Skilling
While AI agents enable automation, over-reliance may erode critical human judgment. Balance is key.
4. Change Management
AI agent deployment often triggers organisational resistance. Clear communication, training, and stakeholder engagement are essential.
The Future of AI Agents: Emerging Trends
- Multi-Agent Systems (MAS) – Collaborative AI agents working together to solve large-scale problems.
- AI Agents with Emotional Intelligence – Enhancing customer service and employee engagement through sentiment-aware interactions.
- Autonomous Enterprise Agents – Entire workflows, from procurement to compliance, managed by autonomous agents.
- Synthetic Colleagues – AI agents acting as digital team members with names, personalities, and specialised functions.
The Executive Imperative
For the C-suite, AI agents are not a futuristic abstraction—they are a present-day imperative. The leaders who understand, embrace, and deploy them effectively will gain enduring advantages in agility, insight, and execution.
To lead in an AI-driven world, executives must move beyond experimentation and pilot programmes. The call to action is clear: invest in strategic AI agent initiatives, build organisational readiness, and embed intelligence into the very DNA of enterprise leadership.
Because in the boardroom of tomorrow, decisions won’t just be made by people—they’ll be shaped by agents designed to make you smarter, faster, and more future-ready than ever before.
🔍 What is Agentic AI?
Agentic AI refers to artificial intelligence systems designed to operate as autonomous agents—capable of perceiving, reasoning, planning, executing tasks, and learning over time to achieve defined objectives, often across complex environments.
The key differentiator is “agency”: the AI not only processes data or performs a single function, but acts purposefully, making independent decisions within a strategic framework—much like a human manager or team member would.
🧠 Core Characteristics of Agentic AI
For the C-Suite, it’s vital to understand what sets Agentic AI apart from traditional AI models:
Feature | Traditional AI Models | Agentic AI Systems |
Task Scope | Narrow (e.g., image classification) | Broad and evolving (e.g., multi-step processes) |
Autonomy | Reactive or supervised | Proactive and goal-driven |
Memory | Stateless or session-based | Persistent memory and learning |
Interactivity | Passive (responds to prompts) | Interactive (plans, initiates, adapts) |
Tool Use | Limited or manual | Can call APIs, search, write code, automate workflows |
Reasoning & Planning | Minimal | Multi-step reasoning and self-correction |
Collaboration | Standalone | Operates in multi-agent ecosystems |
🏢 Why Agentic AI Matters to Enterprises
Agentic AI is not just a technical advancement—it’s a strategic asset. Here’s why it matters to business leaders:
1. Scalable Autonomy
Agentic AI systems can autonomously handle:
- End-to-end business processes (e.g., onboarding, procurement, support)
- Project execution with minimal human intervention
- Real-time decision-making under uncertainty
📌 Imagine an AI agent managing an entire supply chain—from forecasting demand, negotiating with vendors, issuing purchase orders, to tracking logistics. All autonomously.
2. Increased ROI
- Reduces dependency on expensive labour for repetitive knowledge work
- Accelerates task throughput while maintaining quality
- Optimises operational costs through data-driven self-improvement
3. Risk Mitigation
- Detects anomalies and escalates threats in real time (e.g., cybersecurity agents)
- Maintains audit trails and decision logs, improving compliance and governance
- Self-correcting agents reduce operational errors and downtime
🧪 Real-World Examples of Agentic AI in Action
🔹 AutoGPT / BabyAGI
Open-source frameworks that allow users to define a goal, and the agent autonomously breaks it down into sub-tasks, performs web searches, writes code, tests outputs, and refines strategies.
🔹 Enterprise Co-Pilots (Microsoft, Salesforce)
These go beyond chatbots. They proactively summarise meetings, suggest actions, write follow-up emails, and automate CRM updates—functioning like an intelligent assistant with initiative.
🔹 DevOps Agents
AI agents autonomously monitor infrastructure, deploy updates, roll back changes, and scale services based on demand—all without human approval loops.
🔹 Financial Advisory Agents
Used in wealth management, they dynamically adjust portfolios, interpret regulatory changes, and offer tailored investment suggestions, often with direct execution capabilities.
🛠️ Building Blocks of Agentic AI
Understanding the architecture of Agentic AI helps the C-Suite appreciate the required investments:
- LLMs (Large Language Models): e.g., GPT-4, Claude, Gemini
- Memory Architectures: Contextual, episodic, and long-term
- Planning Engines: Goal breakdown, task prioritisation
- Tool Use Interfaces: Plugins, APIs, databases, external software
- Agent Orchestration Frameworks: LangChain, CrewAI, AutoGen
- Governance Modules: Role permissions, guardrails, escalation policies
💡 Think of Agentic AI as the CEO of a digital micro-enterprise—delegating, reporting, iterating, and executing.
AI Agents are autonomous or semi-autonomous systems powered by artificial intelligence that can perceive their environment, reason about it, and act to achieve specific goals—often with little or no human intervention.
🔹 Personal AI Agents
Agent | What it Does |
Siri / Google Assistant / Alexa | Voice assistants that understand natural language, answer questions, control smart devices, and perform tasks like setting alarms or sending texts. |
Replika | An AI companion chatbot that learns and adapts to users’ personalities over time. |
Pi by Inflection AI | A conversational AI that aims to be a personal AI coach, offering emotional support and personalised advice. |
🔹 Productivity & Automation Agents
Agent | What it Does |
AutoGPT / BabyAGI / AgentGPT | LLM-powered agents that can autonomously plan and execute multi-step tasks (e.g., researching, writing reports, updating databases). |
Zapier AI Agents | Automate workflows by taking actions across hundreds of apps based on user instructions or triggers. |
Notion AI | Helps summarise, generate, and rephrase content, functioning as a co-pilot for writing and organisation. |
🔹 Customer Support Agents
Agent | What it Does |
Intercom Fin / Drift AI | Customer service bots that handle FAQs, qualify leads, and escalate issues to human agents. |
ChatGPT in Zendesk / Salesforce | AI that responds to support tickets, drafts replies, or provides suggested answers. |
🔹 Business & Finance Agents
Agent | What it Does |
BloombergGPT | Specialised in financial tasks like market analysis, summarising financial news, and assisting in decision-making. |
Kensho (by S&P Global) | Analyses earnings calls, automates reports, and provides real-time financial insights. |
Numerai Signals | AI agents that submit financial signals for stock trading, rewarded based on accuracy. |
🔹 Healthcare AI Agents
Agent | What it Does |
IBM Watson Health | Analyzes medical literature to assist doctors in diagnosing and suggesting treatments. |
Buoy Health | Conversational AI that guides users to the right care based on symptoms. |
Infermedica | AI-based symptom checker that acts as a triage agent before seeing a doctor. |
🔹 Cybersecurity AI Agents
Agent | What it Does |
Darktrace | Uses AI agents to autonomously detect and respond to cyber threats within a network. |
CrowdStrike Falcon | Endpoint detection agent that proactively hunts threats and stops breaches. |
Microsoft Defender for Endpoint | AI-driven agent that protects devices from malware and network intrusions. |
🔹 Autonomous Systems / Robotics
Agent | What it Does |
Tesla Autopilot | Uses AI to drive cars semi-autonomously by perceiving roads, obstacles, and making real-time driving decisions. |
Boston Dynamics Spot | A robot dog that navigates spaces, collects data, and operates in hazardous environments. |
Amazon Robotics | Autonomous robots in warehouses that manage inventory and optimise delivery workflows. |
🔹 Research & Development Agents
Agent | What it Does |
AlphaFold (by DeepMind) | Predicts 3D protein structures, revolutionising biology research. |
Elicit (by Ought) | AI research assistant that helps with literature review, hypothesis generation, and finding evidence. |
🔐 What are Information Security AI Agents?
They are autonomous or semi-autonomous software systems that use AI/ML to monitor systems, detect anomalies, respond to incidents, and protect digital infrastructure from cyberattacks.
They often fall into one (or more) of these categories:
- Threat Detection
- Vulnerability Management
- Incident Response
- Behavioural Analytics
- Risk Scoring
🔹 Real-World Examples of InfoSec AI Agents
AI Agent | Description | Key Capabilities |
Darktrace Antigena | Self-defending network security agent. | Autonomous response, anomaly detection, ransomware mitigation, works like an “immune system.” |
CrowdStrike Falcon | Endpoint Detection and Response (EDR) agent. | Real-time monitoring, threat hunting, behaviour-based protection, cloud-scale AI. |
Microsoft Defender XDR | AI-powered threat protection across devices and networks. | Cross-domain correlation, automated investigation, auto-remediation. |
Vectra AI Cognito | Detects attacker behaviour across cloud, data centre, and enterprise. | Uses deep learning to track attacker methods, not just signatures. |
SentinelOne Singularity | AI-powered EDR with autonomous detection and remediation. | Storyline™ tech links all related threat activities, isolates infected systems automatically. |
CylancePROTECT (by BlackBerry) | Predictive AI for endpoint security. | Uses machine learning to prevent execution of malicious code, even offline. |
Fortinet FortiAI | On-premises AI agent for threat detection. | Uses deep neural networks to reduce alert fatigue, triage threats, and automate response. |
Elastic Security (Elastic Stack) | AI-powered detection and response platform. | Behavioural analytics, real-time SIEM with machine learning, integrates with threat intel. |
🧠 What Makes These Agents “Smart”?
- Anomaly Detection: Learn what “normal” looks like and flag deviations.
- Natural Language Processing (NLP): Parse logs, security reports, and threat intel feeds.
- Machine Learning: Constantly improves by learning from historical incidents.
- Autonomous Response: Quarantine files, isolate endpoints, revoke credentials—without waiting for human intervention.
- Threat Intelligence Integration: Enriches decisions using feeds like MITRE ATT&CK, VirusTotal, or custom sources.
🎯 Use Cases in Action
- Ransomware Prevention
An AI agent detects file encryption patterns and isolates the affected machine before damage spreads. - Insider Threat Detection
Abnormal data access patterns by employees are flagged for investigation. - Zero-Day Exploit Defence
AI spots unusual traffic behaviour—like a sudden C2 beacon—even if no signature exists yet. - Phishing Attack Response
Emails flagged by users are auto-analysed; malicious ones lead to domain blocking and user alerts. - Automated Threat Hunting
Agents constantly scan logs and system behaviours to uncover hidden attackers or lateral movement.
🔐 How InfoSec AI Agents Add Value
- 24/7 Monitoring: Never sleep, never miss a log line.
- Faster Incident Response: Seconds instead of hours/days.
- Lower False Positives: AI learns to ignore benign events.
- Scalability: Can monitor thousands of endpoints or terabytes of traffic.
🧭 Strategic Considerations for the C-Suite
✅ Opportunities
- Revenue Innovation: Launch new service models with agent-driven operations
- Operational Excellence: Automate complex, multi-departmental processes
- Customer Experience: Deploy personalised agents that learn and evolve per user
⚠️ Risks
- Autonomy Drift: Agents may optimise for unintended metrics or outcomes
- Security: Agents with tool access can be exploited if not properly sandboxed
- Compliance & Explainability: Autonomous decisions must be traceable and auditable
- Change Management: Human teams need support as AI assumes strategic functions
📊 A Maturity Roadmap for Adopting Agentic AI
Stage | Description | Business Impact |
Level 1: Reactive AI | Chatbots, search, classification | Limited automation |
Level 2: Assisted AI | Co-pilots, RPA+AI | Task-level automation |
Level 3: Agentic AI | Goal-driven agents with memory and planning | Process automation |
Level 4: Autonomous AI | Fully autonomous systems with oversight | Organisational transformation |
🔮 The Future of Agentic AI in the Enterprise
We’re entering an era where AI will not just assist—it will act. Expect:
- Digital Executives: AI agents taking on roles like Chief Data Analyst or Compliance Officer
- Agent Swarms: Teams of agents collaborating across domains (e.g., finance, ops, HR)
- Enterprise Operating Systems: Agent-first platforms managing the full digital estate
The challenge for the C-Suite is not whether to adopt Agentic AI, but how quickly and strategically to do so.
🎯 Final Thoughts for Business Leaders
Agentic AI represents a fundamental shift—from machine learning as a service to intelligence as an entity.
- Think of it not as a tool, but as a thinking partner.
- Like human employees, it requires training, governance, and alignment with business values.
- Unlike human employees, it offers limitless scale, 24/7 availability, and exponential learning capacity.
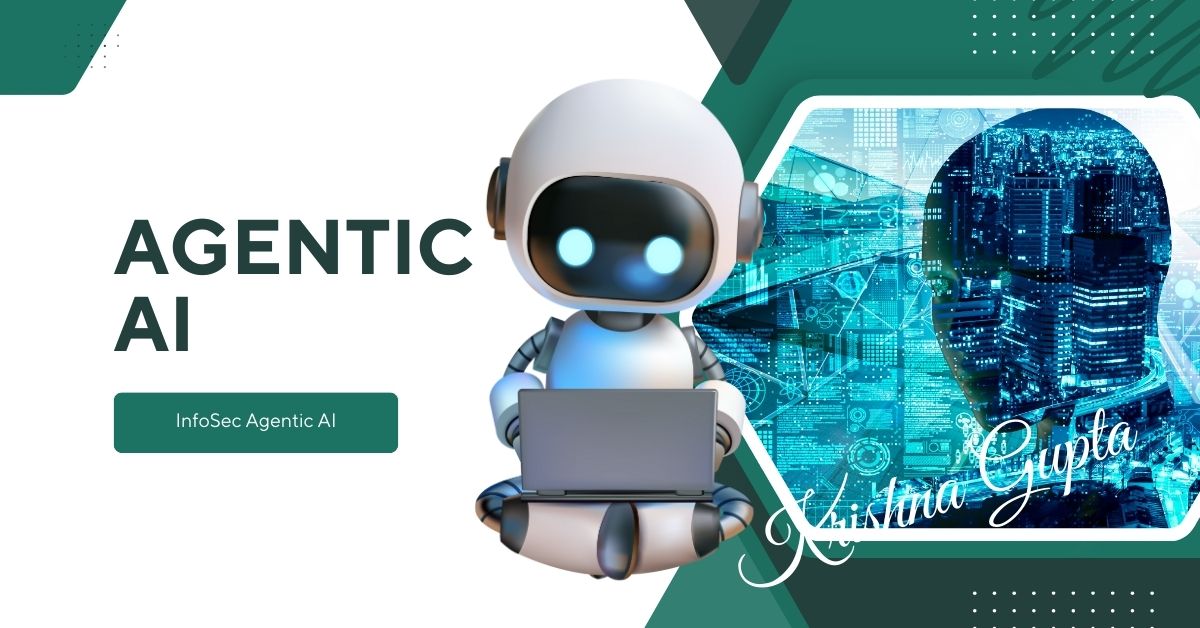
For C-level executives seeking to future-proof their organisation, investing in agentic capabilities today may define your competitive edge tomorrow.