Penetration Testing Anthropic: Securing the Future in an Era of Advanced Cybersecurity Threats
In today’s rapidly evolving cyber landscape, the need for robust security measures has never been greater. With cyber-attacks becoming increasingly sophisticated and the cost of data breaches climbing into the billions, organisations must adopt proactive strategies to safeguard their assets. Penetration testing, often called ethical hacking, is one of the most crucial approaches to identifying and mitigating vulnerabilities before they can be exploited by malicious actors.
However, as the realm of cybersecurity becomes more intricate, the traditional approaches to penetration testing may not be enough. Enter Penetration Testing Anthropic, a concept that seeks to address the dynamic challenges of modern cybersecurity threats by focusing on the human and behavioural aspects of both the attackers and the defenders.
This blog post explores Penetration Testing Anthropic, a new paradigm in ethical hacking, aimed at giving penetration testers, security teams, and C-suite executives a comprehensive understanding of this critical and emerging field. We will delve into its definition, significance, methodologies, and practical applications, providing an insightful analysis and offering actionable advice for navigating the future of cybersecurity.
What is Penetration Testing Anthropic?
Penetration Testing Anthropic combines traditional penetration testing methods with a more nuanced understanding of human behaviour, cognitive psychology, and artificial intelligence (AI). The term “anthropic” refers to anything that relates to human beings or human perspectives, and in this context, it highlights the critical role human elements play in both security and attack strategies.
While traditional penetration testing often focuses on exploiting technical vulnerabilities in systems, Penetration Testing Anthropic goes beyond these boundaries by considering how human behaviours—both of attackers and defenders—can influence the outcome of a cyberattack. This includes social engineering tactics, cognitive biases, organisational culture, decision-making processes, and the integration of AI and machine learning into attack and defence mechanisms.
This approach represents a shift from purely technical penetration testing to a more comprehensive model that accounts for the psychological, social, and technological aspects of cybersecurity.
The Need for Penetration Testing Anthropic
Evolving Threat Landscape
The complexity of modern cyber threats requires a more adaptable and holistic approach to penetration testing. Traditional methods, while effective in identifying vulnerabilities such as open ports, misconfigurations, and unpatched software, often overlook the psychological and behavioural factors that attackers exploit.
A skilled penetration tester today must not only be proficient in technical exploits but also understand how attackers use social engineering techniques to manipulate people and organisational processes. Phishing, pretexting, baiting, and other tactics often target employees, exploiting cognitive biases and emotional responses to gain unauthorised access to sensitive information.
Moreover, with the rise of AI and machine learning, cyber-attacks have become more intelligent, adaptive, and automated. Attackers now use advanced tools to simulate human-like decision-making, conduct surveillance, and adapt to countermeasures. Thus, traditional methods that only focus on technical vulnerabilities fail to address these evolving, multi-dimensional threats.
Human Element in Cybersecurity
Humans remain one of the weakest links in cybersecurity. According to various studies, the majority of security breaches occur due to human error, whether through falling for phishing scams, mismanaging passwords, or neglecting security protocols. Therefore, understanding and testing how human actors interact with security systems is critical for any modern penetration testing approach.
Penetration Testing Anthropic acknowledges this human element by focusing on how attackers manipulate human psychology to bypass security measures. By focusing on the cognitive and emotional responses of individuals within an organisation, this model enables penetration testers to assess not only the strength of technological barriers but also the vulnerabilities within the human chain.
Key Methodologies in Penetration Testing Anthropic
Penetration Testing Anthropic incorporates traditional penetration testing methodologies with a deeper focus on human factors, AI, and behavioural analysis. Below are the key methodologies that characterise this emerging discipline:
1. Social Engineering Attacks
Social engineering attacks manipulate human behaviour to achieve unauthorised access to systems or information. These attacks exploit cognitive biases such as trust, fear, and urgency, which are difficult to defend against using conventional technical measures. Penetration testers working within the anthropic model will incorporate social engineering strategies, including phishing emails, vishing (voice phishing), and impersonation, to test an organisation’s susceptibility to these attacks.
Example: A penetration tester may craft a convincing email that appears to come from an executive or a trusted vendor, asking an employee to click on a malicious link or provide sensitive information. The tester evaluates how well the organisation’s employees adhere to security protocols and detect red flags in these scenarios.
2. Behavioural Modelling and Analysis
Penetration Testing Anthropic uses behavioural psychology to analyse and predict how individuals might act in the event of a security breach. This can include how employees handle suspicious emails, their reactions to an unexpected security alert, or their susceptibility to scams.
Penetration testers can leverage behavioural analysis tools to simulate scenarios and gauge how employees or users interact with the system. This helps identify potential weaknesses in human responses that may be exploited by attackers.
3. Cognitive Bias Testing
Cognitive biases can skew decision-making, and penetration testers working within the anthropic framework will test how such biases impact security. For instance, confirmation bias (where individuals only seek information that supports their existing beliefs) can result in employees dismissing security warnings or ignoring potential threats.
Example: A penetration tester may create a situation where an employee is faced with a situation that aligns with their preconceived notions, like a familiar-looking email that contains a subtle malicious link. The tester would observe whether the employee follows best practices and conducts due diligence before clicking.
4. Artificial Intelligence and Machine Learning Integration
As attackers increasingly use AI and machine learning to adapt and predict security measures, penetration testing must evolve to counter these technologies. AI-driven penetration testing techniques aim to simulate attacks using algorithms that can learn from system responses and adapt their approach in real-time.
AI can also be used to automate the testing of human responses to threats. For example, AI can generate personalised phishing emails or simulate social engineering calls, learning from the responses and evolving its strategies to more accurately mimic real-world threats.
5. Red Teaming and Purple Teaming
Red teaming, which involves simulating realistic attacks to test an organisation’s defence mechanisms, plays a significant role in Penetration Testing Anthropic. Red teamers will use both technical exploits and human-centric attack strategies to evaluate an organisation’s response.
Purple teaming, on the other hand, integrates red and blue teams (offensive and defensive security teams) to work together. This ensures that the human element is tested on both sides: both in the attackers’ strategies and in how defenders identify and respond to these threats.
Real-World Applications of Penetration Testing Anthropic
1. Corporate Espionage Prevention
In industries where intellectual property (IP) is highly valuable, penetration testers using an anthropic approach can identify vulnerabilities in how employees manage sensitive information. This can include weaknesses in how employees share documents, handle proprietary data, or engage in communication that might inadvertently expose valuable secrets.
Penetration testers can also evaluate the human factors in access control, ensuring that individuals with high-level access to IP are following best practices and not falling victim to manipulation.
2. Banking and Financial Services Security
Financial institutions handle vast amounts of personal and financial data, making them prime targets for cybercriminals. In this sector, the anthropic approach to penetration testing is crucial for understanding how human errors can lead to breaches in sensitive data and payment systems.
For example, testers may conduct social engineering tests targeting bank employees to identify how likely they are to disclose client information or perform actions based on false pretenses, such as transferring funds or altering records.
3. Healthcare Cybersecurity
The healthcare industry faces unique challenges when it comes to security. With healthcare professionals constantly under pressure and dealing with sensitive data, human error can significantly impact security. Penetration testers using an anthropic approach can simulate attacks that target medical staff, such as impersonating patients or family members to gain unauthorised access to patient records.
4. Public Sector and Government Security
Penetration Testing Anthropic also plays a vital role in securing government and public sector organisations. These entities often hold sensitive national security information, making them prime targets for cyber espionage and attacks by nation-state actors. Penetration testing that focuses on human behaviour—such as how officials respond to impersonation or phishing attacks—can reveal critical weaknesses that need to be addressed.
Challenges in Implementing Penetration Testing Anthropic
While Penetration Testing Anthropic offers numerous benefits, its implementation presents several challenges:
- Complexity and Resource Intensive: Testing human behaviours and psychological responses requires significant time and effort. It also requires a deep understanding of human psychology and organisational dynamics, which might not always be easy to incorporate into existing testing frameworks.
- Ethical Considerations: Given that Penetration Testing Anthropic involves manipulating human responses and actions, testers must navigate the ethical implications of such testing. Clear guidelines and consent must be established to ensure no harm comes to employees or clients during testing.
- Integration with Traditional Methods: Integrating the anthropic model with traditional technical penetration testing requires careful coordination. While social engineering and human behaviour analysis are essential, technical security measures remain a crucial component of any comprehensive testing strategy.
Penetration Testing the LLM Engines: A New Frontier in Cybersecurity
The advent of large language models (LLMs) such as GPT-3, GPT-4, and other advanced AI systems has revolutionised many sectors, from customer service to content generation, data analysis, and even healthcare. These models, powered by deep learning, have demonstrated remarkable capabilities, including understanding and generating human-like text based on vast datasets.
However, like any complex system, LLM engines are not immune to vulnerabilities. As these AI models become integral to organisational operations, ensuring their security becomes paramount. This leads to the concept of Penetration Testing the LLM Engines—a specialised process aimed at evaluating the security of these advanced AI systems.
In this comprehensive blog post, we will explore the intricacies of penetration testing LLM engines, highlighting the unique challenges, methodologies, and risks associated with these AI systems. This post is designed to provide penetration testers, cybersecurity professionals, and C-suite executives with the necessary insights to secure AI-driven solutions effectively, mitigating risks and enhancing business resilience.
What is Penetration Testing of LLM Engines?
Penetration testing, in its traditional form, is the process of simulating cyber-attacks on an organisation’s IT infrastructure to uncover vulnerabilities that could be exploited by malicious actors. Penetration testing LLM engines takes this concept into the realm of artificial intelligence and machine learning, focusing on assessing and identifying vulnerabilities specific to these AI models and their deployment environments.
The goal of penetration testing LLM engines is to expose weaknesses in the AI’s decision-making processes, interactions with users, and integration into larger systems. These tests aim to identify exploitable vulnerabilities in the model’s training data, algorithms, or API integrations that could be used by attackers to manipulate the model’s behaviour, extract sensitive information, or breach the broader system.
Why is Penetration Testing of LLM Engines Critical?
1. Sensitive Data Exposure
LLMs are often trained on vast amounts of data, including potentially sensitive information. If not properly secured, these models can inadvertently reveal sensitive personal, organisational, or proprietary data through user interactions. Penetration testing LLM engines helps identify data leakage points, ensuring that no unintended information is exposed during model queries.
Example: An LLM might be queried by a user who unknowingly triggers the model to generate private information such as login credentials, medical records, or confidential business strategies. A penetration tester will seek to test and prevent such vulnerabilities.
2. Model Manipulation and Adversarial Attacks
Attackers may attempt to manipulate the output of LLM engines by crafting adversarial inputs—seemingly benign queries that lead the model to produce harmful, biased, or misleading responses. These adversarial attacks could be used to manipulate the model into providing incorrect information, or worse, executing harmful actions if the model is integrated into critical systems.
Example: An attacker could feed an LLM with carefully crafted input that makes the AI generate malicious code or financial advice that exploits system vulnerabilities, potentially resulting in fraud or operational disruptions.
3. Business and Brand Reputation Risks
As organisations increasingly rely on LLM-driven applications (e.g., chatbots, automated writing tools, customer support agents), the integrity of these systems becomes directly linked to the organisation’s reputation. A failure to properly secure the LLM engine can lead to damaging content generation, misinformation, or interactions that harm the brand image.
Example: A customer support bot powered by an LLM might misinterpret a user’s request, generating inappropriate responses that damage customer trust or lead to legal liabilities.
4. AI as a Gateway for Broader Attacks
LLMs are often used as gateways to other systems. For example, an LLM integrated into an organisation’s internal network could be exploited to bypass security measures, gain access to sensitive databases, or manipulate other AI systems. Penetration testing can help identify such weaknesses before they are exploited by attackers.
Key Areas of Focus for Penetration Testing LLM Engines
1. Training Data Vulnerabilities
One of the core components of LLMs is their training data. These models are trained on massive datasets that are often scraped from the internet, encompassing a broad range of topics, including both useful and potentially harmful information.
Penetration testers should assess whether the LLM has been exposed to any harmful, biased, or incorrect data that could lead to the generation of malicious outputs. Additionally, testers should explore how well the model handles edge cases or ambiguous inputs that could result in errors or data leakage.
Key Test Areas:
- Data Poisoning: Testing for vulnerabilities in the model’s training data, such as the inclusion of deliberately inserted harmful data designed to skew the model’s behaviour.
- Biases and Discriminatory Outputs: Evaluating the model’s responses for bias or discriminatory content, which can pose both ethical and legal risks.
2. Input Manipulation (Adversarial Testing)
Adversarial testing of LLMs involves crafting inputs that subtly manipulate the model’s outputs, often in ways that are undetectable by normal users. These manipulations can exploit weaknesses in the AI’s decision-making process and result in outputs that are damaging to the user or organisation.
Penetration testers will need to develop adversarial strategies, such as:
- Prompt Injection: Manipulating a model’s responses by injecting specific phrases or keywords into user prompts.
- Poisoning Attacks: Feeding the model with specific adversarial queries designed to degrade its performance or cause it to make erroneous or harmful decisions.
Example: An attacker could input a request to an LLM that triggers a faulty decision-making process, causing the AI to generate illegal or unsafe recommendations.
3. API and Integration Security
Many LLM engines are accessible via APIs that interact with other systems, applications, and platforms. These APIs are often vulnerable points of attack, particularly if they are not properly secured or if access controls are weak.
Penetration testers must assess the security of the APIs through which LLMs are accessed, focusing on:
- Authentication and Authorisation: Ensuring only authorised users can access the LLM’s functionality.
- Data Integrity: Verifying that the input and output data between the LLM and its integrators are encrypted and that the API is resistant to common attacks such as SQL injection or Cross-Site Scripting (XSS).
- Rate Limiting and Input Validation: Ensuring that the model’s API can handle large volumes of requests without being overwhelmed or manipulated by malicious actors.
4. Output Poisoning and Content Filtering
Output poisoning refers to the intentional manipulation of an LLM’s generated content to include harmful or malicious information. This could include the model generating fake news, defamation, or harmful advice. Effective output filtering mechanisms are critical to mitigate this risk.
Penetration testers should:
- Evaluate the effectiveness of filters that prevent the model from producing harmful content.
- Test whether the LLM could be tricked into bypassing these filters using adversarial inputs or hidden prompts.
Example: An attacker could craft a series of queries designed to make the LLM output racist or sexist responses that harm an organisation’s public image.
5. Ethical and Privacy Considerations
Given the potential risks associated with LLMs, penetration testers must also consider ethical and privacy concerns. These models often handle sensitive user data, and improper handling could lead to significant privacy violations. Testers should evaluate:
- Data Retention: Ensuring that personal data is not retained by the model after a session ends.
- User Consent: Verifying that the model’s interactions are transparent and that users understand what data is being collected and how it is used.
Methodologies for Penetration Testing LLM Engines
Penetration testing LLM engines follows similar principles as traditional penetration testing but with an emphasis on understanding AI-specific risks. Below are common methodologies:
1. Black-box Testing
In black-box testing, penetration testers evaluate the LLM engine without knowledge of its internal workings. This method simulates an attack from an external actor and focuses on how the system responds to various inputs.
2. White-box Testing
White-box testing, on the other hand, involves full access to the model’s architecture, training data, and underlying algorithms. This allows testers to explore potential vulnerabilities within the model itself, including flaws in the training data or weaknesses in the model’s design.
3. Red Teaming
A red team performs simulated attacks from a perspective of a real-world adversary. For LLM engines, this would involve simulating adversarial input generation, social engineering, and manipulation tactics that could compromise the AI’s outputs.
4. Fuzz Testing
Fuzz testing involves inputting a large number of random or semi-random data into the LLM to find weaknesses in its processing mechanisms. It is particularly useful in identifying edge cases or situations where the model’s response is unpredictable or flawed.
Final Thoughts
Penetration testing LLM engines is an emerging field in cybersecurity, driven by the increasing reliance on AI technologies across all industries. As AI models grow in complexity and ubiquity, ensuring their security is vital for organisations that rely on them for critical operations. From adversarial attacks to privacy concerns, LLM engines present unique challenges that demand specialised testing techniques. By adopting comprehensive penetration testing strategies, organisations can identify vulnerabilities in these systems before they can be exploited, safeguarding both their reputation and their customers’ trust.
As the capabilities of LLM engines continue to evolve, so too must our understanding of how they can be manipulated or exploited. Penetration testing these models will remain a critical task for cybersecurity professionals in the years ahead, ensuring that AI-driven technologies are as secure as they are innovative.
Penetration Testing Anthropic is a forward-thinking approach that enhances traditional penetration testing by incorporating human and behavioural factors. With cyber-attacks becoming increasingly sophisticated, organisations can no longer rely solely on technical defences. To build truly resilient systems, it is essential to account for the human element and adapt to the emerging threats posed by AI and machine learning.
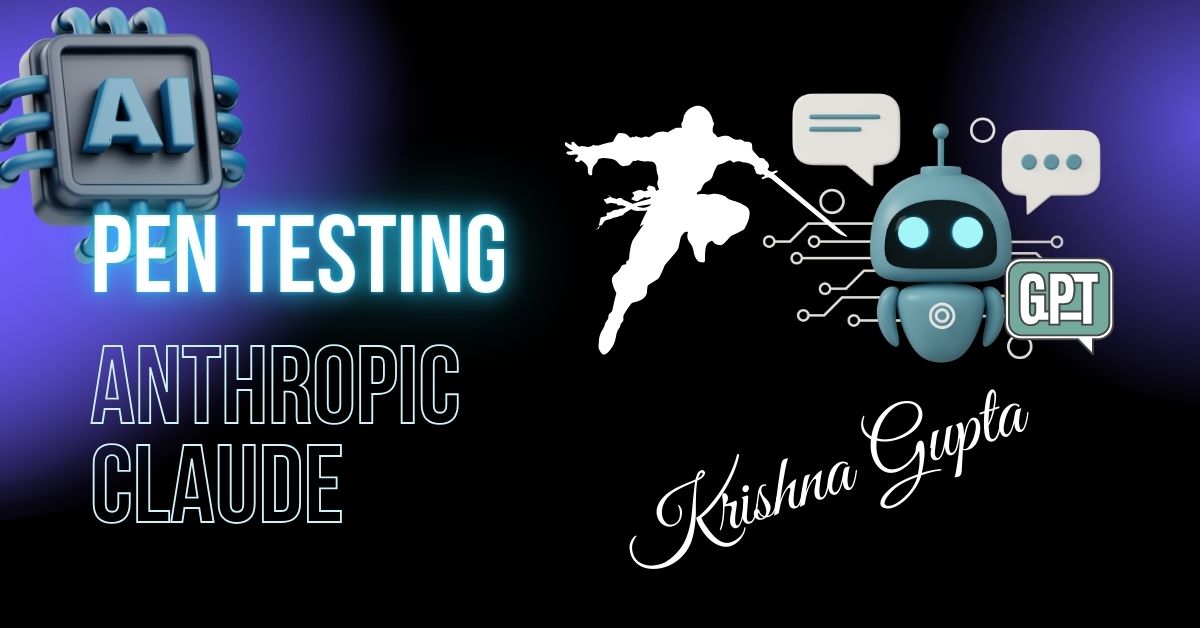
By adopting an anthropic approach to penetration testing, organisations can better understand the vulnerabilities that exist not only in their technology but also in their people and processes. For penetration testers, this shift offers exciting new